Healthcare Transparency: An Analysis of State-Level Transparency Regulations' Cost Effects in American Hospitals
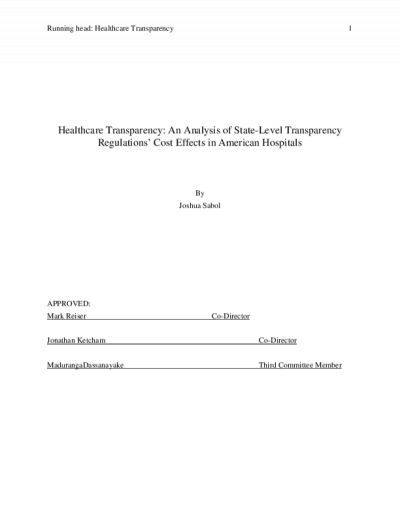
Description
Objective: To assess and quantify the effect of state’s price transparency regulations (hereafter, PTR) on healthcare pricing.
Data Sources: I use the Healthcare Cost and Utilization Project’s Nationwide Inpatient Sample (NIS) from 2000 to 2011. The NIS is a 20% sample of all inpatient claims. The Manhattan Institute supplied data on the availability of health savings accounts in each state. State PTR implementation dates were gathered by Hans Christensen, Eric Floyd, and Mark Maffett of University of Chicago’s Booth School of Business by contacting the health department, hospital association, or website controller in each state.
Study Design: The NIS data was collapsed by procedure, hospital, and year providing averages for the dependent variable, Cost, and a host of covariates. Cost is a product of Total Charges within the NIS and the hospital’s Cost to Charge ratio. A new binary variable, PTR, was defined as ‘0’ if the year was strictly less than the disclosure website’s implementation date, ‘1’ for afterwards, and missing for the year of implementation. Then, using multivariate OLS regression with fixed effect modeling, the change in cost from before to after the year of implementation is estimated.
Principal Findings: The analysis estimates the effect of PTR to decrease the average cost per procedure by 7%. Specifications identify within state, within hospital, and within procedure variation, and reports that 78% of the cost decrease is due to within-hospital, within-procedure price discounts. An additional model includes the interaction of PTR with the prevalence of health savings accounts (hereafter, HSAs) and procedure electivity. The results show that PTR lowers costs by an additional 3 percent with each additional 10 percentage point increase in the availability of HSAs. In contrast, the cost reductions from PTR were much smaller for procedures more frequently coded as elective.
Conclusions: The study concludes price transparency regulations can lead to a decrease in a procedure’s costs on average, primarily through price discounts and slightly through lower cost procedures, but not due to patients moving to cheaper hospitals. This implies that hospitals are taking initiative and lowering prices as the competition’s prices become publically available suggesting that hospitals – not patients – are the biggest users of price transparency websites. Hospitals are also finding some ways to provide cheaper alternatives to more expensive procedures. State regulators should evaluate if a better metric other than charge prices, such as expected out-of-pocket payments, would evoke greater patient participation. Furthermore, states with higher prevalence of HSAs experience greater effects of PTR as expected since patients with HSAs have greater incentives to lower their costs. Patients should expect a shift towards plans that offer these types of savings accounts since they’ve shown to have a reduction of health costs on average per procedure in states with higher prevalence of HSAs.
Data Sources: I use the Healthcare Cost and Utilization Project’s Nationwide Inpatient Sample (NIS) from 2000 to 2011. The NIS is a 20% sample of all inpatient claims. The Manhattan Institute supplied data on the availability of health savings accounts in each state. State PTR implementation dates were gathered by Hans Christensen, Eric Floyd, and Mark Maffett of University of Chicago’s Booth School of Business by contacting the health department, hospital association, or website controller in each state.
Study Design: The NIS data was collapsed by procedure, hospital, and year providing averages for the dependent variable, Cost, and a host of covariates. Cost is a product of Total Charges within the NIS and the hospital’s Cost to Charge ratio. A new binary variable, PTR, was defined as ‘0’ if the year was strictly less than the disclosure website’s implementation date, ‘1’ for afterwards, and missing for the year of implementation. Then, using multivariate OLS regression with fixed effect modeling, the change in cost from before to after the year of implementation is estimated.
Principal Findings: The analysis estimates the effect of PTR to decrease the average cost per procedure by 7%. Specifications identify within state, within hospital, and within procedure variation, and reports that 78% of the cost decrease is due to within-hospital, within-procedure price discounts. An additional model includes the interaction of PTR with the prevalence of health savings accounts (hereafter, HSAs) and procedure electivity. The results show that PTR lowers costs by an additional 3 percent with each additional 10 percentage point increase in the availability of HSAs. In contrast, the cost reductions from PTR were much smaller for procedures more frequently coded as elective.
Conclusions: The study concludes price transparency regulations can lead to a decrease in a procedure’s costs on average, primarily through price discounts and slightly through lower cost procedures, but not due to patients moving to cheaper hospitals. This implies that hospitals are taking initiative and lowering prices as the competition’s prices become publically available suggesting that hospitals – not patients – are the biggest users of price transparency websites. Hospitals are also finding some ways to provide cheaper alternatives to more expensive procedures. State regulators should evaluate if a better metric other than charge prices, such as expected out-of-pocket payments, would evoke greater patient participation. Furthermore, states with higher prevalence of HSAs experience greater effects of PTR as expected since patients with HSAs have greater incentives to lower their costs. Patients should expect a shift towards plans that offer these types of savings accounts since they’ve shown to have a reduction of health costs on average per procedure in states with higher prevalence of HSAs.
Date Created
The date the item was original created (prior to any relationship with the ASU Digital Repositories.)
2015-05
Agent
- Author (aut): Sabol, Joshua Lawrence
- Thesis director: Reiser, Mark
- Committee member: Ketcham, Jonathan
- Committee member: Dassanayake, Maduranga
- Contributor (ctb): Barrett, The Honors College
- Contributor (ctb): School of Mathematical and Statistical Sciences
- Contributor (ctb): Department of Supply Chain Management