Using Facebook to Examine Smoking Behavior through ""Quit Smoking"" Support Groups
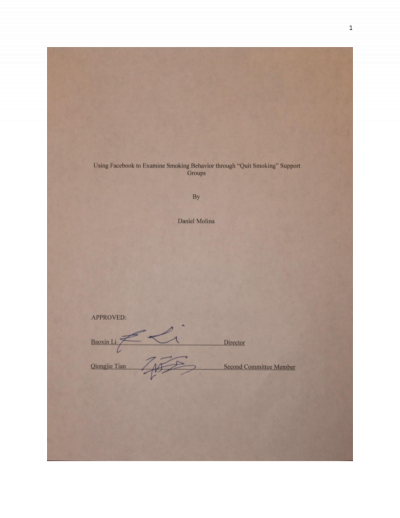
Description
Background: As the growth of social media platforms continues, the use of the constantly increasing amount of freely available, user-generated data they receive becomes of great importance. One apparent use of this content is public health surveillance; such as for increasing understanding of substance abuse. In this study, Facebook was used to monitor nicotine addiction through the public support groups users can join to aid their quitting process. Objective: The main objective of this project was to gain a better understanding of the mechanisms of nicotine addiction online and provide content analysis of Facebook posts obtained from "quit smoking" support groups. Methods: Using the Facebook Application Programming Interface (API) for Python, a sample of 9,970 posts were collected in October 2015. Information regarding the user's name and the number of likes and comments they received on their post were also included. The posts crawled were then manually classified by one annotator into one of three categories: positive, negative, and neutral. Where positive posts are those that describe current quits, negative posts are those that discuss relapsing, and neutral posts are those that were not be used to train the classifiers, which include posts where users have yet to attempt a quit, ads, random questions, etc. For this project, the performance of two machine learning algorithms on a corpus of manually labeled Facebook posts were compared. The classification goal was to test the plausibility of creating a natural language processing machine learning classifier which could be used to distinguish between relapse (labeled negative) and quitting success (labeled positive) posts from a set of smoking related posts. Results: From the corpus of 9,970 posts that were manually labeled: 6,254 (62.7%) were labeled positive, 1,249 (12.5%) were labeled negative, and 2467 (24.8%) were labeled neutral. Since the posts labeled neutral are those which are irrelevant to the classification task, 7,503 posts were used to train the classifiers: 83.4% positive and 16.6% negative. The SVM classifier was 84.1% accurate and 84.1% precise, had a recall of 1, and an F-score of 0.914. The MNB classifier was 82.8% accurate and 82.8% precise, had a recall of 1, and an F-score of 0.906. Conclusions: From the Facebook surveillance results, a small peak is given into the behavior of those looking to quit smoking. Ultimately, what makes Facebook a great tool for public health surveillance is that it has an extremely large and diverse user base with information that is easily obtainable. This, and the fact that so many people are actually willing to use Facebook support groups to aid their quitting processes demonstrates that it can be used to learn a lot about quitting and smoking behavior.
Date Created
The date the item was original created (prior to any relationship with the ASU Digital Repositories.)
2016-05
Agent
- Author (aut): Molina, Daniel Antonio
- Thesis director: Li, Baoxin
- Committee member: Tian, Qiongjie
- Contributor (ctb): School of Mathematical and Statistical Sciences
- Contributor (ctb): Barrett, The Honors College