The Analysis of Customer Lifetime Value: How to Segment and Measure the Segmentation Accuracy
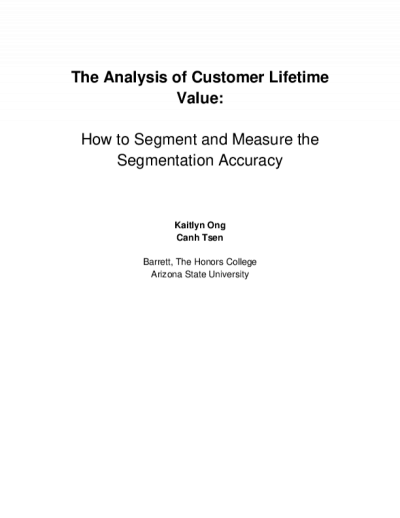
Description
Customer lifetime value has been a popular topic within the marketing field with which many researchers and marketing managers have been dwelling. The topic plays an important role in customer segmentation and has been studied and applied in a variety of business areas. The main objective of customer lifetime value and customer segmentation is to classify the importance level of each client to a company and compare it to other clients. Questions, such as which marketing strategies should be implemented for which customers and how much should be invested in a certain group of customers can all be answered by customer lifetime value and customer segmentation. However, the related literature is missing comparative research on assessing the amount of time from the initial point of acquisition that a client needs to be with the company in order to accurately predict its customer segment. This paper intends to provide a clarification to the problem. Purpose: Analyze customer profitability with clustering analysis and identify how many years does a customer need to be with a company to accurately predict its customer segment. By determining this number, managers can understand their clients better and establish which clients will most likely yield a greater profit at an early stage of the relationship. Methodology: Using data mining to clean and prepare our financial services dataset, we selected young clients who were less than ten years old. Linear regression and K-means clustering analyses then returned five clusters of clients. Next, we predicted the accuracy levels of customers with two to seven data points against the "correct" segment. Lastly, we validated our overall prediction accuracy levels using the chance probability and the desired classification accuracy, calculated from a discriminant analysis. Findings: We found that using five data points or more to cluster returned percentage accuracies greater than the desired classification accuracy. However, this desired classification accuracy and the percentage accuracies were fairly low and not sufficient to use as a base for business decisions and other managerial purposes.
Date Created
The date the item was original created (prior to any relationship with the ASU Digital Repositories.)
2016-12
Agent
- Co-author: Ong, Kaitlyn
- Co-author: Tsen, Canh
- Thesis director: Hollmann, Thomas
- Committee member: Han, Sang Pil
- Contributor (ctb): Department of Information Systems
- Contributor (ctb): Department of Marketing
- Contributor (ctb): Barrett, The Honors College