Data and Predictive Analytics for Energy Use
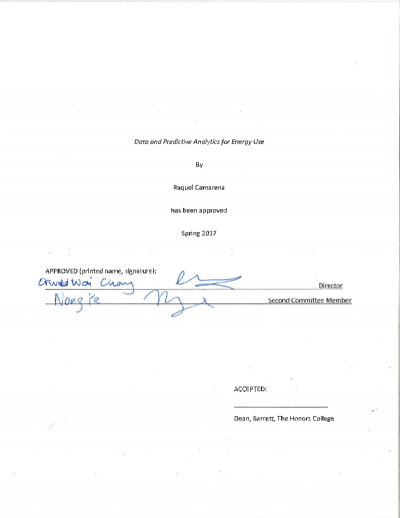
Description
The overall energy consumption around the United States has not been reduced even with the advancement of technology over the past decades. Deficiencies exist between design and actual energy performances. Energy Infrastructure Systems (EIS) are impacted when the amount of energy production cannot be accurately and efficiently forecasted. Inaccurate engineering assumptions can result when there is a lack of understanding on how energy systems can operate in real-world applications. Energy systems are complex, which results in unknown system behaviors, due to an unknown structural system model. Currently, there exists a lack of data mining techniques in reverse engineering, which are needed to develop efficient structural system models. In this project, a new type of reverse engineering algorithm has been applied to a year's worth of energy data collected from an ASU research building called MacroTechnology Works, to identify the structural system model. Developing and understanding structural system models is the first step in creating accurate predictive analytics for energy production. The associative network of the building's data will be highlighted to accurately depict the structural model. This structural model will enhance energy infrastructure systems' energy efficiency, reduce energy waste, and narrow the gaps between energy infrastructure design, planning, operation and management (DPOM).
Date Created
The date the item was original created (prior to any relationship with the ASU Digital Repositories.)
2016-12
Agent
- Author (aut): Camarena, Raquel Jimenez
- Thesis director: Chong, Oswald
- Committee member: Ye, Nong
- Contributor (ctb): Industrial, Systems
- Contributor (ctb): Barrett, The Honors College