Neoantigen Prediction Pipeline
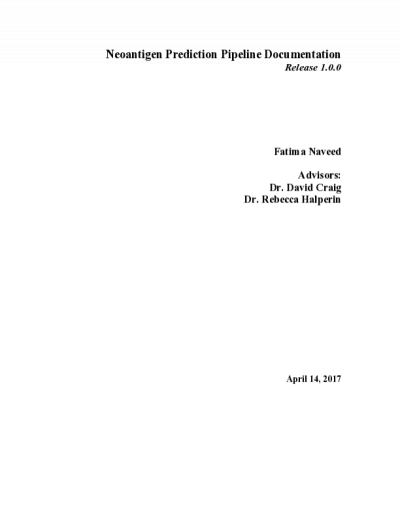
Description
Cells become cancerous due to changes in their genetic makeup. In cancers, an altered amino acid due to a tumor mutation can result in proteins that are identified as "foreign" by the immune system. An MHC molecule will bind to these "foreign" peptide fragments, also called neoantigens. There are 2 classes of MHC molecules. While the MHC I complex is found in all cells with a nucleus, MHC II complexes are mostly found in antigen presenting cells (APCs), such as macrophages, B cells, and dendritic cells. The MHC molecule then presents the neoantigen on the cell's surface. If an immune cell, such as a T-cell, is able to bind to the neoantigen, it can then destroy the tumor cell. However, there are molecules that act as checkpoints on certain immune cells that have to be activated or inactivated to start an immune response. This ensures that healthy cells are not being killed. However, sometimes cancer cells can find ways to use these checkpoints to avoid being attacked. An example of immunotherapy which has had clinical successes is checkpoint blockade inhibition, which means blocking the activity of immune checkpoint proteins in order to release the "brakes" on the immune system to increase its ability to destroy cancer cells. Studies have found that there is a correlation between mutational load and response to immunotherapy. The goal of this project is to create a pipeline that identifies tumor neoantigens. This involved researching various softwares and implementing them to work together. This project involved developing a neoantigen prediction pipeline, which works with TGen's genomics pipeline, to help understand a patient's immune response. The neoantigen prediction pipeline first creates two protein fastas from the high quality non-synonymous mutations, frameshifts, codon insertions, and codon deletions from vcfmerger. One of the protein fastas includes the mutations, while the other one does not representing the wildtype protein. The pipeline then predicts both classes of HLA genotypes of the MHC molecules using DNA or RNA expression in the form of fastqs. The protein fastas and each HLA are fed into IEDB to obtain peptide-MHC binding predictions. Wildtype peptides and neoantigens with low binding affinities are then removed. RNA expression information is then added into the final text file from dseq and sailfish files from TGen's genomics pipeline.
Date Created
The date the item was original created (prior to any relationship with the ASU Digital Repositories.)
2017-05
Agent
- Author (aut): Naveed, Fatima
- Thesis director: Craig, David
- Committee member: Halperin, Rebecca
- Contributor (ctb): Computer Science and Engineering Program
- Contributor (ctb): Computer Science and Engineering Program
- Contributor (ctb): Barrett, The Honors College