Assessing cognitive learning of analytical problem solving
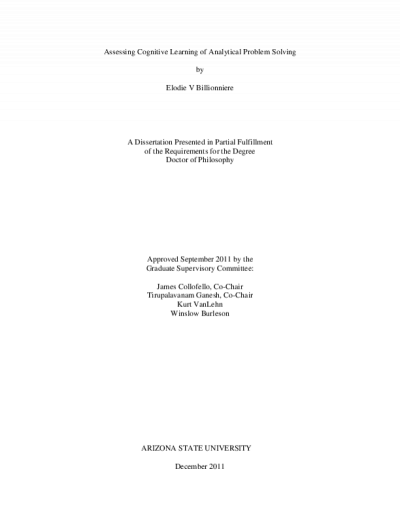
Description
Introductory programming courses, also known as CS1, have a specific set of expected outcomes related to the learning of the most basic and essential computational concepts in computer science (CS). However, two of the most often heard complaints in such courses are that (1) they are divorced from the reality of application and (2) they make the learning of the basic concepts tedious. The concepts introduced in CS1 courses are highly abstract and not easily comprehensible. In general, the difficulty is intrinsic to the field of computing, often described as "too mathematical or too abstract." This dissertation presents a small-scale mixed method study conducted during the fall 2009 semester of CS1 courses at Arizona State University. This study explored and assessed students' comprehension of three core computational concepts - abstraction, arrays of objects, and inheritance - in both algorithm design and problem solving. Through this investigation students' profiles were categorized based on their scores and based on their mistakes categorized into instances of five computational thinking concepts: abstraction, algorithm, scalability, linguistics, and reasoning. It was shown that even though the notion of computational thinking is not explicit in the curriculum, participants possessed and/or developed this skill through the learning and application of the CS1 core concepts. Furthermore, problem-solving experiences had a direct impact on participants' knowledge skills, explanation skills, and confidence. Implications for teaching CS1 and for future research are also considered.
Date Created
The date the item was original created (prior to any relationship with the ASU Digital Repositories.)
2011
Agent
- Author (aut): Billionniere, Elodie V
- Thesis advisor (ths): Collofello, James
- Thesis advisor (ths): Ganesh, Tirupalavanam G.
- Committee member: VanLehn, Kurt
- Committee member: Burleson, Winslow
- Publisher (pbl): Arizona State University