Educational Data Mining: An Application of a Predictive Model of Online Student Enrollment Decisions
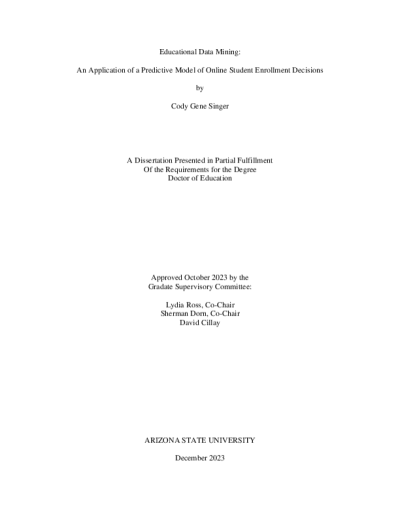
Description
College and university enrollment has decreased nationwide every year for more than a decade as educational consumers increasingly question the value of higher education and discover alternatives to the traditional university system. Enrollment professionals seeking growth are tasked to develop and implement innovative solutions to address increasing enrollment challenges by being responsive to consumer values, interests, and needs. This multi-phase mixed methods action research study explores educational data mining and machine learning to understand and predict the enrollment decisions of admitted applicants (n=3,843) at the online campus of a public research university (phase one). Then, this innovation is distributed to understand how university enrollment professionals (n=7) interpret and are affected by the factors that influence online student enrollment decisions (phase two). Logistic regression was used to evaluate 24 independent variables to classify each applicant into a dichotomous dependent outcome: will an applicant enroll or will they not. The model identified 10 significant predictors and accurately categorized 81% of the enrollment outcomes at its peak. The population was comprised of online adult learners and the findings were carefully compared to the findings of previous studies which differed in institutional settings (on campus) and student populations (first-year students). Additionally, the study aimed to extend the work of previous literature through a second application phase within the local context. The second phase was guided by distributed leadership theory and the four-stage theory of organizational change and introduced the model to enrollment professionals within the local context through participation in a workshop coupled with a pre-/post-workshop survey. This convergent parallel mixed methods design resulted in themes that demonstrated enrollment managers had a genuine desire to understand and apply the model to assist in solving complex enrollment challenges and were interested in using the model to inform their perspectives, decision-making, and strategy development. This study concludes that educational data mining and machine learning can be used to predict the enrollment decisions of online adult students and that enrollment managers can use the data to inform the many enrollment challenges they are tasked to overcome.
Date Created
The date the item was original created (prior to any relationship with the ASU Digital Repositories.)
2023
Agent
- Author (aut): Singer, Cody Gene
- Thesis advisor (ths): Ross, Lydia
- Thesis advisor (ths): Dorn, Sherman
- Committee member: Cillay, David
- Publisher (pbl): Arizona State University