Virality in the Digital Age: Contextualization, Messaging Strategies, and Framing Detection
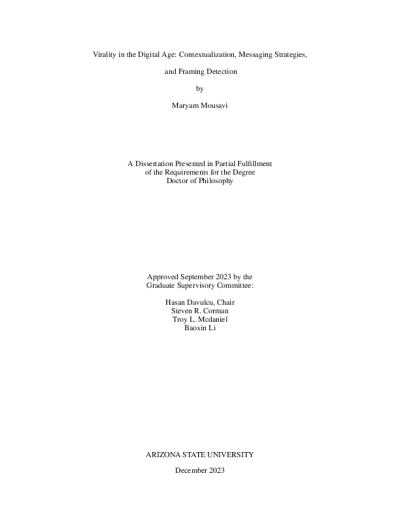
Description
Social networking platforms have redefined communication, serving as conduits forswift global information dissemination on contemporary topics and trends. This research
probes information cascade (IC) dynamics, focusing on viral IC, where user-shared information
gains rapid, widespread attention. Implications of IC span advertising, persuasion,
opinion-shaping, and crisis response.
First, this dissertation aims to unravel the context behind viral content, particularly in
the realm of the digital world, introducing a semi-supervised taxonomy induction framework
(STIF). STIF employs state-of-the-art term representation, topical phrase detection,
and clustering to organize terms into a two-level topic taxonomy. Social scientists then
assess the topic clusters for coherence and completeness. STIF proves effective, significantly
reducing human coding efforts (up to 74%) while accurately inducing taxonomies
and term-to-topic mappings due to the high purity of its topics. Second, to profile the
drivers of virality, this study investigates messaging strategies influencing message virality.
Three content-based hypotheses are formulated and tested, demonstrating that incorporation
of “negativity bias,” “causal arguments,” and “threats to personal or societal core
values” - singularly and jointly - significantly enhances message virality on social media,
quantified by retweet counts. Furthermore, the study highlights framing narratives’ pivotal
role in shaping discourse, particularly in adversarial campaigns. An innovative pipeline
for automatic framing detection is introduced, and tested on a collection of texts on the
Russia-Ukraine conflict. Integrating representation learning, overlapping graph-clustering,
and a unique Topic Actor Graph (TAG) synthesis method, the study achieves remarkable
framing detection accuracy. The developed scoring mechanism maps sentences to automatically
detect framing signatures. This pipeline attains an impressive F1 score of 92%
and a 95% weighted accuracy for framing detection on a real-world dataset.
In essence, this dissertation focuses on the multidimensional exploration of information cascade, uncovering the context and drivers of content virality, and automating framing detection.
Through innovative methodologies like STIF, messaging strategy analysis, and
TAG Frames, the research contributes valuable insights into the mechanics of viral content
spread and framing nuances within the digital landscape, enriching fields such as advertisement,
communication, public discourse, and crisis response strategies.
Date Created
The date the item was original created (prior to any relationship with the ASU Digital Repositories.)
2023
Agent
- Author (aut): Mousavi, Maryam
- Thesis advisor (ths): Davulcu, Hasan HD
- Committee member: Li, Baoxin
- Committee member: Corman, Steven
- Committee member: McDaniel, Troy
- Publisher (pbl): Arizona State University