Correlated Scenario Generation Using Generative Models
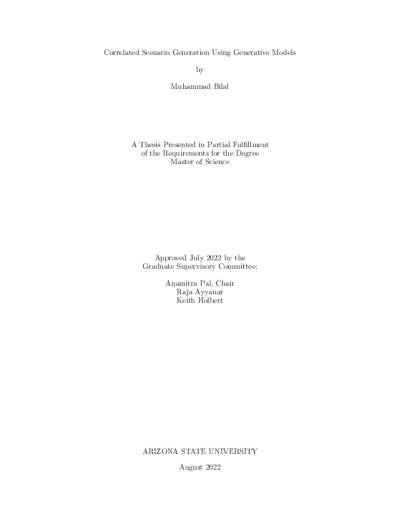
Description
With the continued increase in the amount of renewable generation in the formof distributed energy resources, reliability planning has progressively become a more
challenging task for the modern power system. This is because with higher penetration
of renewable generation, the system has to bear a higher degree of variability
and uncertainty. One way to address this problem is by generating realistic scenarios
that complement and supplement actual system conditions. This thesis presents
a methodology to create such correlated synthetic scenarios for load and renewable
generation using machine learning.
Machine learning algorithms need to have ample amounts of data available to
them for training purposes. However, real-world datasets are often skewed in the
distribution of the different events in the sample space. Data augmentation and
scenario generation techniques are often utilized to complement the datasets with
additional samples or by filling in missing data points. Datasets pertaining to the
electric power system are especially prone to having very few samples for certain
events, such as abnormal operating conditions, as they are not very common in an
actual power system. A recurrent generative adversarial network (GAN) model is
presented in this thesis to generate solar and load scenarios in a correlated manner
using an actual dataset obtained from a power utility located in the U.S. Southwest.
The generated solar and load profiles are verified both statistically and by implementation
on a simulated test system, and the performance of correlated scenario
generation vs. uncorrelated scenario generation is investigated. Given the interconnected
relationships between the variables of the dataset, it is observed that correlated
scenario generation results in more realistic synthetic scenarios, particularly for abnormal
system conditions. When combined with actual but scarce abnormal conditions,
the augmented dataset of system conditions provides a better platform for performing contingency studies for a more thorough reliability planning.
The proposed scenario generation method is scalable and can be modified to work
with different time-series datasets. Moreover, when the model is trained in a conditional
manner, it can be used to synthesise any number of scenarios for the different
events present in a given dataset. In summary, this thesis explores scenario generation
using a recurrent conditional GAN and investigates the benefits of correlated
generation compared to uncorrelated synthesis of profiles for the reliability planning
problem of power systems.
Date Created
The date the item was original created (prior to any relationship with the ASU Digital Repositories.)
2022
Agent
- Author (aut): Bilal, Muhammad
- Thesis advisor (ths): Pal, Anamitra
- Committee member: Holbert, Keith
- Committee member: Ayyanar, Raja
- Publisher (pbl): Arizona State University