Computational Accounts of Trust in Human AI Interaction
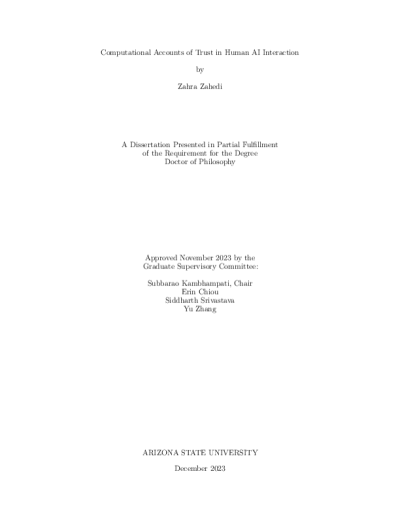
Description
The growing presence of AI-driven systems in everyday life calls for the development of efficient methods to facilitate interactions between humans and AI agents. At the heart of these interactions lies the notion of trust, a key element shaping human behavior and decision-making. It is essential to foster a suitable level of trust to ensure the success of human-AI collaborations, while recognizing that excessive or misplaced trust can lead to unfavorable consequences. Human-AI partnerships face distinct hurdles, particularly potential misunderstandings about AI capabilities. This emphasizes the need for AI agents to better understand and adjust human expectations and trust. The thesis explores the dynamics of trust in human-robot interactions, acknowledging that the term encompasses human-AI interactions, and emphasizes the importance of understanding trust in these relationships. This thesis first presents a mental model-based framework that contextualizes trust in human-AI interactions, capturing multi-faceted dimensions often overlooked in computational trust studies. Then, I use this framework as a basis for developing decision-making frameworks that incorporate trust in both single and longitudinal human-AI interactions. Finally, this mental model-based framework enables the inference and estimation of trust when direct measures are not feasible.
Date Created
The date the item was original created (prior to any relationship with the ASU Digital Repositories.)
2023
Agent
- Author (aut): Zahedi, Zahra
- Thesis advisor (ths): Kambhampati, Subbarao SK
- Committee member: Chiou, Erin EC
- Committee member: Srivastava, Siddharth SS
- Committee member: Zhang, Yu YZ
- Publisher (pbl): Arizona State University