Bayesian-Entropy Method for Probabilistic Diagnostics and Prognostics of Engineering Systems
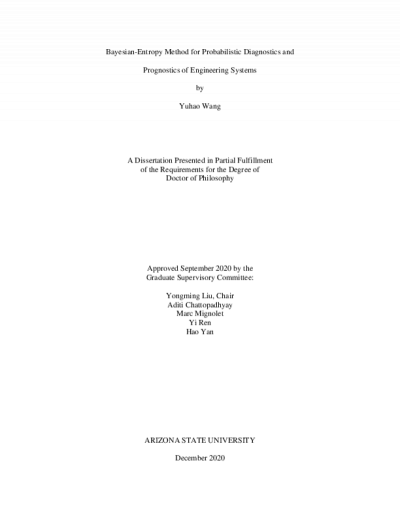
Description
Information exists in various forms and a better utilization of the available information can benefit the system awareness and response predictions. The focus of this dissertation is on the fusion of different types of information using Bayesian-Entropy method. The Maximum Entropy method in information theory introduces a unique way of handling information in the form of constraints. The Bayesian-Entropy (BE) principle is proposed to integrate the Bayes’ theorem and Maximum Entropy method to encode extra information. The posterior distribution in Bayesian-Entropy method has a Bayesian part to handle point observation data, and an Entropy part that encodes constraints, such as statistical moment information, range information and general function between variables. The proposed method is then extended to its network format as Bayesian Entropy Network (BEN), which serves as a generalized information fusion tool for diagnostics, prognostics, and surrogate modeling.
The proposed BEN is demonstrated and validated with extensive engineering applications. The BEN method is first demonstrated for diagnostics of gas pipelines and metal/composite plates for damage diagnostics. Both empirical knowledge and physics model are integrated with direct observations to improve the accuracy for diagnostics and to reduce the training samples. Next, the BEN is demonstrated in prognostics and safety assessment in air traffic management system. Various information types, such as human concepts, variable correlation functions, physical constraints, and tendency data, are fused in BEN to enhance the safety assessment and risk prediction in the National Airspace System (NAS). Following this, the BE principle is applied in surrogate modeling. Multiple algorithms are proposed based on different type of information encoding, such as Bayesian-Entropy Linear Regression (BELR), Bayesian-Entropy Semiparametric Gaussian Process (BESGP), and Bayesian-Entropy Gaussian Process (BEGP) are demonstrated with numerical toy problems and practical engineering analysis. The results show that the major benefits are the superior prediction/extrapolation performance and significant reduction of training samples by using additional physics/knowledge as constraints. The proposed BEN offers a systematic and rigorous way to incorporate various information sources. Several major conclusions are drawn based on the proposed study.
The proposed BEN is demonstrated and validated with extensive engineering applications. The BEN method is first demonstrated for diagnostics of gas pipelines and metal/composite plates for damage diagnostics. Both empirical knowledge and physics model are integrated with direct observations to improve the accuracy for diagnostics and to reduce the training samples. Next, the BEN is demonstrated in prognostics and safety assessment in air traffic management system. Various information types, such as human concepts, variable correlation functions, physical constraints, and tendency data, are fused in BEN to enhance the safety assessment and risk prediction in the National Airspace System (NAS). Following this, the BE principle is applied in surrogate modeling. Multiple algorithms are proposed based on different type of information encoding, such as Bayesian-Entropy Linear Regression (BELR), Bayesian-Entropy Semiparametric Gaussian Process (BESGP), and Bayesian-Entropy Gaussian Process (BEGP) are demonstrated with numerical toy problems and practical engineering analysis. The results show that the major benefits are the superior prediction/extrapolation performance and significant reduction of training samples by using additional physics/knowledge as constraints. The proposed BEN offers a systematic and rigorous way to incorporate various information sources. Several major conclusions are drawn based on the proposed study.
Date Created
The date the item was original created (prior to any relationship with the ASU Digital Repositories.)
2020
Agent
- Author (aut): Wang, Yuhao
- Thesis advisor (ths): Liu, Yongming
- Committee member: Chattopadhyay, Aditi
- Committee member: Mignolet, Marc
- Committee member: Yan, Hao
- Committee member: Ren, Yi
- Publisher (pbl): Arizona State University