Self-supervised Representation Learning via Image Out-painting for Medical Image Analysis
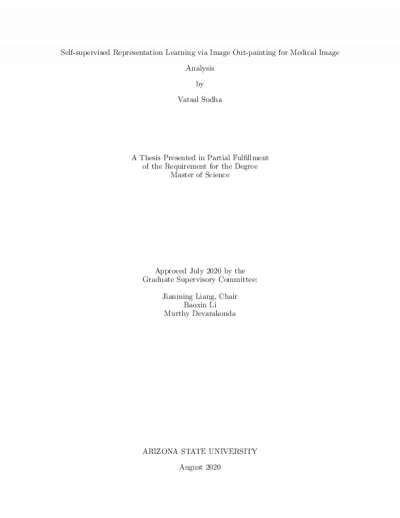
Description
In recent years, Convolutional Neural Networks (CNNs) have been widely used in not only the computer vision community but also within the medical imaging community. Specifically, the use of pre-trained CNNs on large-scale datasets (e.g., ImageNet) via transfer learning for a variety of medical imaging applications, has become the de facto standard within both communities.
However, to fit the current paradigm, 3D imaging tasks have to be reformulated and solved in 2D, losing rich 3D contextual information. Moreover, pre-trained models on natural images never see any biomedical images and do not have knowledge about anatomical structures present in medical images. To overcome the above limitations, this thesis proposes an image out-painting self-supervised proxy task to develop pre-trained models directly from medical images without utilizing systematic annotations. The idea is to randomly mask an image and train the model to predict the missing region. It is demonstrated that by predicting missing anatomical structures when seeing only parts of the image, the model will learn generic representation yielding better performance on various medical imaging applications via transfer learning.
The extensive experiments demonstrate that the proposed proxy task outperforms training from scratch in six out of seven medical imaging applications covering 2D and 3D classification and segmentation. Moreover, image out-painting proxy task offers competitive performance to state-of-the-art models pre-trained on ImageNet and other self-supervised baselines such as in-painting. Owing to its outstanding performance, out-painting is utilized as one of the self-supervised proxy tasks to provide generic 3D pre-trained models for medical image analysis.
However, to fit the current paradigm, 3D imaging tasks have to be reformulated and solved in 2D, losing rich 3D contextual information. Moreover, pre-trained models on natural images never see any biomedical images and do not have knowledge about anatomical structures present in medical images. To overcome the above limitations, this thesis proposes an image out-painting self-supervised proxy task to develop pre-trained models directly from medical images without utilizing systematic annotations. The idea is to randomly mask an image and train the model to predict the missing region. It is demonstrated that by predicting missing anatomical structures when seeing only parts of the image, the model will learn generic representation yielding better performance on various medical imaging applications via transfer learning.
The extensive experiments demonstrate that the proposed proxy task outperforms training from scratch in six out of seven medical imaging applications covering 2D and 3D classification and segmentation. Moreover, image out-painting proxy task offers competitive performance to state-of-the-art models pre-trained on ImageNet and other self-supervised baselines such as in-painting. Owing to its outstanding performance, out-painting is utilized as one of the self-supervised proxy tasks to provide generic 3D pre-trained models for medical image analysis.
Date Created
The date the item was original created (prior to any relationship with the ASU Digital Repositories.)
2020
Agent
- Author (aut): Sodha, Vatsal Arvindkumar
- Thesis advisor (ths): Liang, Jianming
- Committee member: Devarakonda, Murthy
- Committee member: Li, Baoxin
- Publisher (pbl): Arizona State University