You and I are Not the Same: A Comparison of Human and Artificial Intelligent Advisors
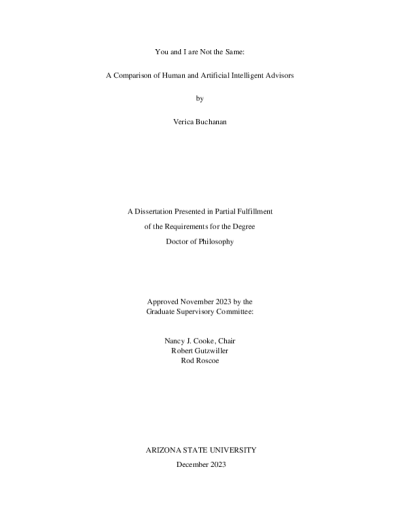
Description
It is difficult to imagine a society that does not utilize teams. At the same time, teams need to evolve to meet today’s challenges of the ever-increasing proliferation of data and complexity. It may be useful to add artificial intelligent (AI) agents to team up with humans. Then, as AI agents are integrated into the team, the first study asks what roles can AI agents take? The first study investigates this issue by asking whether an AI agent can take the role of a facilitator and in turn, improve planning outcomes by facilitating team processes. Results indicate that the human facilitator was significantly better than the AI facilitator at reducing cognitive biases such as groupthink, anchoring, and information pooling, as well as increasing decision quality and score. Additionally, participants viewed the AI facilitator negatively and ignored its inputs compared to the human facilitator. Yet, participants in the AI Facilitator condition performed significantly better than participants in the No Facilitator condition, illustrating that having an AI facilitator was better than having no facilitator at all. The second study explores whether artificial social intelligence (ASI) agents can take the role of advisors and subsequently improve team processes and mission outcome during a simulated search-and-rescue mission. The results of this study indicate that although ASI advisors can successfully advise teams, they also use a significantly greater number of taskwork interventions than teamwork interventions. Additionally, this study served to identify what the ASI advisors got right compared to the human advisor and vice versa. Implications and future directions are discussed.
Date Created
The date the item was original created (prior to any relationship with the ASU Digital Repositories.)
2023
Agent
- Author (aut): Buchanan, Verica
- Thesis advisor (ths): Cooke, Nancy J.
- Committee member: Gutzwiller, Robert S.
- Committee member: Roscoe, Rod D.
- Publisher (pbl): Arizona State University