Data Driven Inference in Populations of Agents
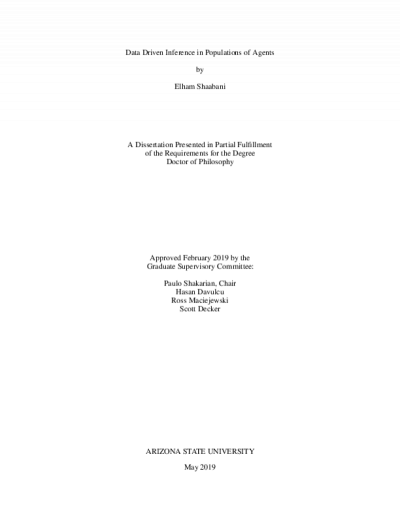
Description
In the artificial intelligence literature, three forms of reasoning are commonly employed to understand agent behavior: inductive, deductive, and abductive. More recently, data-driven approaches leveraging ideas such as machine learning, data mining, and social network analysis have gained popularity. While data-driven variants of the aforementioned forms of reasoning have been applied separately, there is little work on how data-driven approaches across all three forms relate and lend themselves to practical applications. Given an agent behavior and the percept sequence, how one can identify a specific outcome such as the likeliest explanation? To address real-world problems, it is vital to understand the different types of reasonings which can lead to better data-driven inference.
This dissertation has laid the groundwork for studying these relationships and applying them to three real-world problems. In criminal modeling, inductive and deductive reasonings are applied to early prediction of violent criminal gang members. To address this problem the features derived from the co-arrestee social network as well as geographical and temporal features are leveraged. Then, a data-driven variant of geospatial abductive inference is studied in missing person problem to locate the missing person. Finally, induction and abduction reasonings are studied for identifying pathogenic accounts of a cascade in social networks.
This dissertation has laid the groundwork for studying these relationships and applying them to three real-world problems. In criminal modeling, inductive and deductive reasonings are applied to early prediction of violent criminal gang members. To address this problem the features derived from the co-arrestee social network as well as geographical and temporal features are leveraged. Then, a data-driven variant of geospatial abductive inference is studied in missing person problem to locate the missing person. Finally, induction and abduction reasonings are studied for identifying pathogenic accounts of a cascade in social networks.
Date Created
The date the item was original created (prior to any relationship with the ASU Digital Repositories.)
2019
Agent
- Author (aut): Shaabani, Elham
- Thesis advisor (ths): Shakarian, Paulo
- Committee member: Davulcu, Hasan
- Committee member: Maciejewski, Ross
- Committee member: Decker, Scott
- Publisher (pbl): Arizona State University