Human Activity Recognition and Control of Wearable Robots
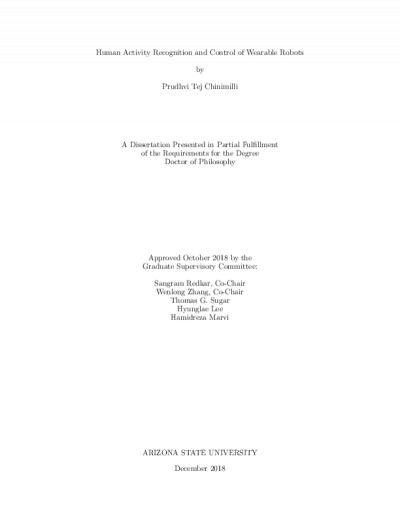
Description
Wearable robotics has gained huge popularity in recent years due to its wide applications in rehabilitation, military, and industrial fields. The weakness of the skeletal muscles in the aging population and neurological injuries such as stroke and spinal cord injuries seriously limit the abilities of these individuals to perform daily activities. Therefore, there is an increasing attention in the development of wearable robots to assist the elderly and patients with disabilities for motion assistance and rehabilitation. In military and industrial sectors, wearable robots can increase the productivity of workers and soldiers. It is important for the wearable robots to maintain smooth interaction with the user while evolving in complex environments with minimum effort from the user. Therefore, the recognition of the user's activities such as walking or jogging in real time becomes essential to provide appropriate assistance based on the activity.
This dissertation proposes two real-time human activity recognition algorithms intelligent fuzzy inference (IFI) algorithm and Amplitude omega ($A \omega$) algorithm to identify the human activities, i.e., stationary and locomotion activities. The IFI algorithm uses knee angle and ground contact forces (GCFs) measurements from four inertial measurement units (IMUs) and a pair of smart shoes. Whereas, the $A \omega$ algorithm is based on thigh angle measurements from a single IMU.
This dissertation also attempts to address the problem of online tuning of virtual impedance for an assistive robot based on real-time gait and activity measurement data to personalize the assistance for different users. An automatic impedance tuning (AIT) approach is presented for a knee assistive device (KAD) in which the IFI algorithm is used for real-time activity measurements. This dissertation also proposes an adaptive oscillator method known as amplitude omega adaptive oscillator ($A\omega AO$) method for HeSA (hip exoskeleton for superior augmentation) to provide bilateral hip assistance during human locomotion activities. The $A \omega$ algorithm is integrated into the adaptive oscillator method to make the approach robust for different locomotion activities. Experiments are performed on healthy subjects to validate the efficacy of the human activities recognition algorithms and control strategies proposed in this dissertation. Both the activity recognition algorithms exhibited higher classification accuracy with less update time. The results of AIT demonstrated that the KAD assistive torque was smoother and EMG signal of Vastus Medialis is reduced, compared to constant impedance and finite state machine approaches. The $A\omega AO$ method showed real-time learning of the locomotion activities signals for three healthy subjects while wearing HeSA. To understand the influence of the assistive devices on the inherent dynamic gait stability of the human, stability analysis is performed. For this, the stability metrics derived from dynamical systems theory are used to evaluate unilateral knee assistance applied to the healthy participants.
This dissertation proposes two real-time human activity recognition algorithms intelligent fuzzy inference (IFI) algorithm and Amplitude omega ($A \omega$) algorithm to identify the human activities, i.e., stationary and locomotion activities. The IFI algorithm uses knee angle and ground contact forces (GCFs) measurements from four inertial measurement units (IMUs) and a pair of smart shoes. Whereas, the $A \omega$ algorithm is based on thigh angle measurements from a single IMU.
This dissertation also attempts to address the problem of online tuning of virtual impedance for an assistive robot based on real-time gait and activity measurement data to personalize the assistance for different users. An automatic impedance tuning (AIT) approach is presented for a knee assistive device (KAD) in which the IFI algorithm is used for real-time activity measurements. This dissertation also proposes an adaptive oscillator method known as amplitude omega adaptive oscillator ($A\omega AO$) method for HeSA (hip exoskeleton for superior augmentation) to provide bilateral hip assistance during human locomotion activities. The $A \omega$ algorithm is integrated into the adaptive oscillator method to make the approach robust for different locomotion activities. Experiments are performed on healthy subjects to validate the efficacy of the human activities recognition algorithms and control strategies proposed in this dissertation. Both the activity recognition algorithms exhibited higher classification accuracy with less update time. The results of AIT demonstrated that the KAD assistive torque was smoother and EMG signal of Vastus Medialis is reduced, compared to constant impedance and finite state machine approaches. The $A\omega AO$ method showed real-time learning of the locomotion activities signals for three healthy subjects while wearing HeSA. To understand the influence of the assistive devices on the inherent dynamic gait stability of the human, stability analysis is performed. For this, the stability metrics derived from dynamical systems theory are used to evaluate unilateral knee assistance applied to the healthy participants.
Date Created
The date the item was original created (prior to any relationship with the ASU Digital Repositories.)
2018
Agent
- Author (aut): Chinimilli, Prudhvi Tej
- Thesis advisor (ths): Redkar, Sangram
- Thesis advisor (ths): Zhang, Wenlong
- Committee member: Sugar, Thomas G.
- Committee member: Lee, Hyunglae
- Committee member: Marvi, Hamidreza
- Publisher (pbl): Arizona State University