A Framework for Spatial Database Explanations
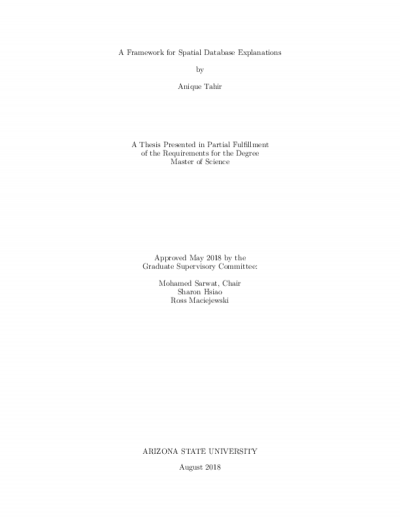
Description
In the last few years, there has been a tremendous increase in the use of big data. Most of this data is hard to understand because of its size and dimensions. The importance of this problem can be emphasized by the fact that Big Data Research and Development Initiative was announced by the United States administration in 2012 to address problems faced by the government. Various states and cities in the US gather spatial data about incidents like police calls for service.
When we query large amounts of data, it may lead to a lot of questions. For example, when we look at arithmetic relationships between queries in heterogeneous data, there are a lot of differences. How can we explain what factors account for these differences? If we define the observation as an arithmetic relationship between queries, this kind of problem can be solved by aggravation or intervention. Aggravation views the value of our observation for different set of tuples while intervention looks at the value of the observation after removing sets of tuples. We call the predicates which represent these tuples, explanations. Observations by themselves have limited importance. For example, if we observe a large number of taxi trips in a specific area, we might ask the question: Why are there so many trips here? Explanations attempt to answer these kinds of questions.
While aggravation and intervention are designed for non spatial data, we propose a new approach for explaining spatially heterogeneous data. Our approach expands on aggravation and intervention while using spatial partitioning/clustering to improve explanations for spatial data. Our proposed approach was evaluated against a real-world taxi dataset as well as a synthetic disease outbreak datasets. The approach was found to outperform aggravation in precision and recall while outperforming intervention in precision.
When we query large amounts of data, it may lead to a lot of questions. For example, when we look at arithmetic relationships between queries in heterogeneous data, there are a lot of differences. How can we explain what factors account for these differences? If we define the observation as an arithmetic relationship between queries, this kind of problem can be solved by aggravation or intervention. Aggravation views the value of our observation for different set of tuples while intervention looks at the value of the observation after removing sets of tuples. We call the predicates which represent these tuples, explanations. Observations by themselves have limited importance. For example, if we observe a large number of taxi trips in a specific area, we might ask the question: Why are there so many trips here? Explanations attempt to answer these kinds of questions.
While aggravation and intervention are designed for non spatial data, we propose a new approach for explaining spatially heterogeneous data. Our approach expands on aggravation and intervention while using spatial partitioning/clustering to improve explanations for spatial data. Our proposed approach was evaluated against a real-world taxi dataset as well as a synthetic disease outbreak datasets. The approach was found to outperform aggravation in precision and recall while outperforming intervention in precision.
Date Created
The date the item was original created (prior to any relationship with the ASU Digital Repositories.)
2018
Agent
- Author (aut): Tahir, Anique
- Thesis advisor (ths): Elsayed, Mohamed
- Committee member: Hsiao, Ihan
- Committee member: Maciejewski, Ross
- Publisher (pbl): Arizona State University