Sequencing Effects and Loss Aversion in a Delay Discounting Task
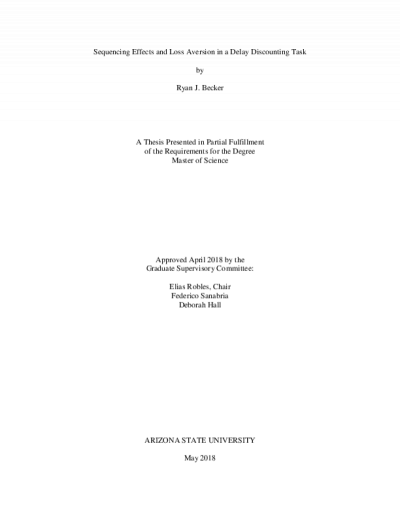
Description
The attractiveness of a reward depends in part on the delay to its receipt, with more distant rewards generally being valued less than more proximate ones. The rate at which people discount the value of delayed rewards has been associated with a variety of clinically and socially relevant human behaviors. Thus, the accurate measurement of delay discounting rates is crucial to the study of mechanisms underlying behaviors such as risky sex, addiction, and gambling. In delay discounting tasks, participants make choices between two alternatives: one small amount of money delivered immediately versus a large amount of money delivered after a delay. After many choices, the experimental task will converge on an indifference point: the value of the delayed reward that approximates the value of the immediate one. It has been shown that these indifference points are systematically biased by the direction in which one of the alternatives adjusts. This bias is termed a sequencing effect.
The present research proposed a reference-dependent model of choice drawn from Prospect Theory to account for the presence of sequencing effects in a delay discounting task. Sensitivity to reference frames and sequencing effects were measured in two computer tasks. Bayesian and frequentist analyses indicated that the reference-dependent model of choice cannot account for sequencing effects. Thus, an alternative, perceptual account of sequencing effects that draws on a Bayesian framework of magnitude estimation is proposed and furnished with some preliminary evidence. Implications for future research in the measurement of delay discounting and sensitivity to reference frames are discussed.
The present research proposed a reference-dependent model of choice drawn from Prospect Theory to account for the presence of sequencing effects in a delay discounting task. Sensitivity to reference frames and sequencing effects were measured in two computer tasks. Bayesian and frequentist analyses indicated that the reference-dependent model of choice cannot account for sequencing effects. Thus, an alternative, perceptual account of sequencing effects that draws on a Bayesian framework of magnitude estimation is proposed and furnished with some preliminary evidence. Implications for future research in the measurement of delay discounting and sensitivity to reference frames are discussed.
Date Created
The date the item was original created (prior to any relationship with the ASU Digital Repositories.)
2018
Agent
- Author (aut): Becker, Ryan J
- Thesis advisor (ths): Robles, Elías
- Committee member: Sanabria, Federico
- Committee member: Hall, Deborah L.
- Publisher (pbl): Arizona State University