Anomaly detection in categorical datasets with artificial contrasts
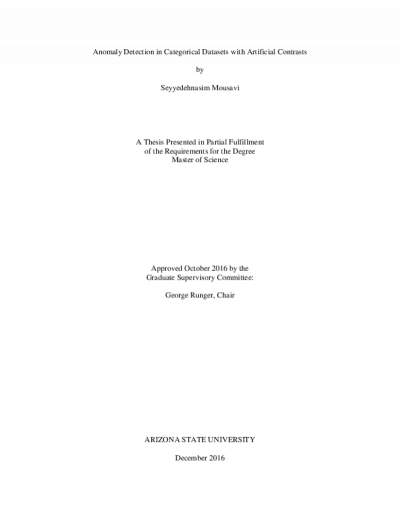
Description
Anomaly is a deviation from the normal behavior of the system and anomaly detection techniques try to identify unusual instances based on deviation from the normal data. In this work, I propose a machine-learning algorithm, referred to as Artificial Contrasts, for anomaly detection in categorical data in which neither the dimension, the specific attributes involved, nor the form of the pattern is known a priori. I use RandomForest (RF) technique as an effective learner for artificial contrast. RF is a powerful algorithm that can handle relations of attributes in high dimensional data and detect anomalies while providing probability estimates for risk decisions.
I apply the model to two simulated data sets and one real data set. The model was able to detect anomalies with a very high accuracy. Finally, by comparing the proposed model with other models in the literature, I demonstrate superior performance of the proposed model.
I apply the model to two simulated data sets and one real data set. The model was able to detect anomalies with a very high accuracy. Finally, by comparing the proposed model with other models in the literature, I demonstrate superior performance of the proposed model.
Date Created
The date the item was original created (prior to any relationship with the ASU Digital Repositories.)
2016
Agent
- Author (aut): Mousavi, Seyyedehnasim
- Thesis advisor (ths): Runger, George C.
- Committee member: Wu, Teresa
- Committee member: Kim, Sunghoon
- Publisher (pbl): Arizona State University