TiCTak: target-specific centrality manipulation on large networks
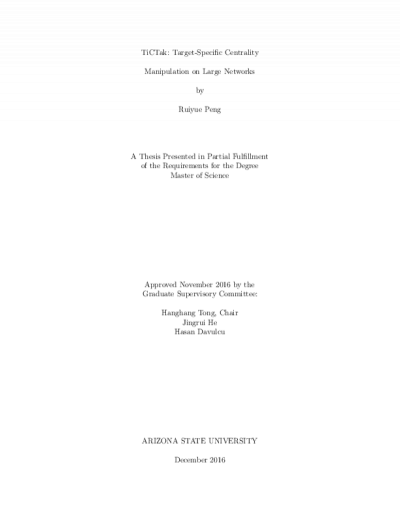
Description
Measuring node centrality is a critical common denominator behind many important graph mining tasks. While the existing literature offers a wealth of different node centrality measures, it remains a daunting task on how to intervene the node centrality in a desired way. In this thesis, we study the problem of minimizing the centrality of one or more target nodes by edge operation. The heart of the proposed method is an accurate and efficient algorithm to estimate the impact of edge deletion on the spectrum of the underlying network, based on the observation that the edge deletion is essentially a local, sparse perturbation to the original network. Extensive experiments are conducted on a diverse set of real networks to demonstrate the effectiveness, efficiency and scalability of our approach. In particular, it is average of 260.95%, in terms of minimizing eigen-centrality, better than the standard matrix-perturbation based algorithm, with lower time complexity.
Date Created
The date the item was original created (prior to any relationship with the ASU Digital Repositories.)
2016
Agent
- Author (aut): Peng, Ruiyue
- Thesis advisor (ths): Tong, Hanghang
- Committee member: He, Jingrui
- Committee member: Davulcu, Hasan
- Publisher (pbl): Arizona State University