Look-ahead information based optimization strategy for hybrid electric vehicles
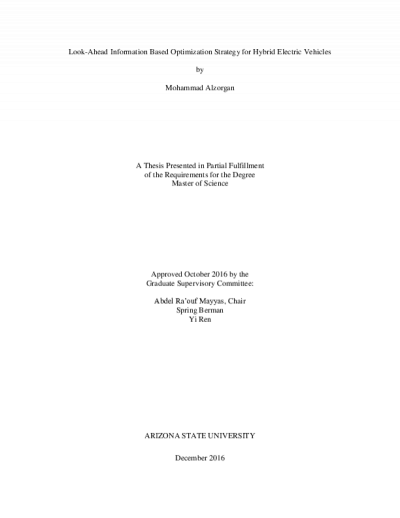
Description
The environmental impact of the fossil fuels has increased tremendously in the last decade. This impact is one of the most contributing factors of global warming. This research aims to reduce the amount of fuel consumed by vehicles through optimizing the control scheme for the future route information. Taking advantage of more degrees of freedom available within PHEV, HEV, and FCHEV “energy management” allows more margin to maximize efficiency in the propulsion systems. The application focuses on reducing the energy consumption in vehicles by acquiring information about the road grade. Road elevations are obtained by use of Geographic Information System (GIS) maps to optimize the controller. The optimization is then reflected on the powertrain of the vehicle.The approach uses a Model Predictive Control (MPC) algorithm that allows the energy management strategy to leverage road grade to prepare the vehicle for minimizing energy consumption during an uphill and potential energy harvesting during a downhill. The control algorithm will predict future energy/power requirements of the vehicle and optimize the performance by instructing the power split between the internal combustion engine (ICE) and the electric-drive system. Allowing for more efficient operation and higher performance of the PHEV, and HEV. Implementation of different strategies, such as MPC and Dynamic Programming (DP), is considered for optimizing energy management systems. These strategies are utilized to have a low processing time. This approach allows the optimization to be integrated with ADAS applications, using current technology for implementable real time applications.
The Thesis presents multiple control strategies designed, implemented, and tested using real-world road elevation data from three different routes. Initial simulation based results show significant energy savings. The savings range between 11.84% and 25.5% for both Rule Based (RB) and DP strategies on the real world tested routes. Future work will take advantage of vehicle connectivity and ADAS systems to utilize Vehicle to Vehicle (V2V), Vehicle to Infrastructure (V2I), traffic information, and sensor fusion to further optimize the PHEV and HEV toward more energy efficient operation.
The Thesis presents multiple control strategies designed, implemented, and tested using real-world road elevation data from three different routes. Initial simulation based results show significant energy savings. The savings range between 11.84% and 25.5% for both Rule Based (RB) and DP strategies on the real world tested routes. Future work will take advantage of vehicle connectivity and ADAS systems to utilize Vehicle to Vehicle (V2V), Vehicle to Infrastructure (V2I), traffic information, and sensor fusion to further optimize the PHEV and HEV toward more energy efficient operation.
Date Created
The date the item was original created (prior to any relationship with the ASU Digital Repositories.)
2016
Agent
- Author (aut): Alzorgan, Mohammad
- Thesis advisor (ths): Mayyas, Abdel Ra’ouf
- Committee member: Berman, Spring
- Committee member: Ren, Yi
- Publisher (pbl): Arizona State University