Expanding data mining theory for industrial applications
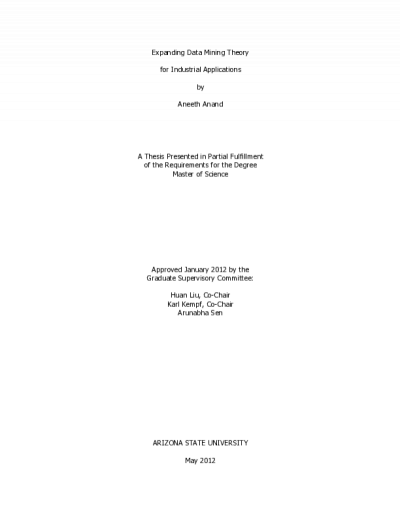
Description
The field of Data Mining is widely recognized and accepted for its applications in many business problems to guide decision-making processes based on data. However, in recent times, the scope of these problems has swollen and the methods are under scrutiny for applicability and relevance to real-world circumstances. At the crossroads of innovation and standards, it is important to examine and understand whether the current theoretical methods for industrial applications (which include KDD, SEMMA and CRISP-DM) encompass all possible scenarios that could arise in practical situations. Do the methods require changes or enhancements? As part of the thesis I study the current methods and delineate the ideas of these methods and illuminate their shortcomings which posed challenges during practical implementation. Based on the experiments conducted and the research carried out, I propose an approach which illustrates the business problems with higher accuracy and provides a broader view of the process. It is then applied to different case studies highlighting the different aspects to this approach.
Date Created
The date the item was original created (prior to any relationship with the ASU Digital Repositories.)
2012
Agent
- Author (aut): Anand, Aneeth
- Thesis advisor (ths): Liu, Huan
- Thesis advisor (ths): Kempf, Karl G.
- Committee member: Sen, Arunabha
- Publisher (pbl): Arizona State University