Brain dynamics based automated epileptic seizure detection
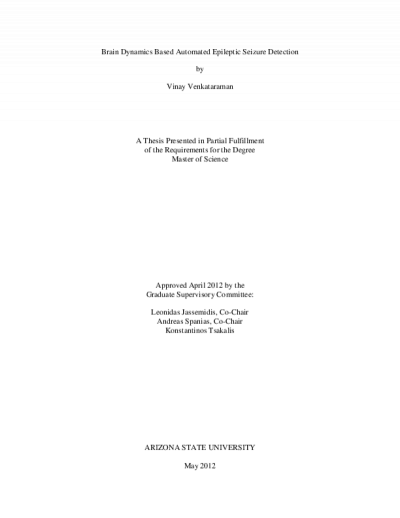
Description
Approximately 1% of the world population suffers from epilepsy. Continuous long-term electroencephalographic (EEG) monitoring is the gold-standard for recording epileptic seizures and assisting in the diagnosis and treatment of patients with epilepsy. However, this process still requires that seizures are visually detected and marked by experienced and trained electroencephalographers. The motivation for the development of an automated seizure detection algorithm in this research was to assist physicians in such a laborious, time consuming and expensive task. Seizures in the EEG vary in duration (seconds to minutes), morphology and severity (clinical to subclinical, occurrence rate) within the same patient and across patients. The task of seizure detection is also made difficult due to the presence of movement and other recording artifacts. An early approach towards the development of automated seizure detection algorithms utilizing both EEG changes and clinical manifestations resulted to a sensitivity of 70-80% and 1 false detection per hour. Approaches based on artificial neural networks have improved the detection performance at the cost of algorithm's training. Measures of nonlinear dynamics, such as Lyapunov exponents, have been applied successfully to seizure prediction. Within the framework of this MS research, a seizure detection algorithm based on measures of linear and nonlinear dynamics, i.e., the adaptive short-term maximum Lyapunov exponent (ASTLmax) and the adaptive Teager energy (ATE) was developed and tested. The algorithm was tested on long-term (0.5-11.7 days) continuous EEG recordings from five patients (3 with intracranial and 2 with scalp EEG) and a total of 56 seizures, producing a mean sensitivity of 93% and mean specificity of 0.048 false positives per hour. The developed seizure detection algorithm is data-adaptive, training-free and patient-independent. It is expected that this algorithm will assist physicians in reducing the time spent on detecting seizures, lead to faster and more accurate diagnosis, better evaluation of treatment, and possibly to better treatments if it is incorporated on-line and real-time with advanced neuromodulation therapies for epilepsy.
Date Created
The date the item was original created (prior to any relationship with the ASU Digital Repositories.)
2012
Agent
- Author (aut): Venkataraman, Vinay
- Thesis advisor (ths): Jassemidis, Leonidas
- Thesis advisor (ths): Spanias, Andreas
- Committee member: Tsakalis, Konstantinos
- Publisher (pbl): Arizona State University