Generalized linear models in Bayesian phylogeography
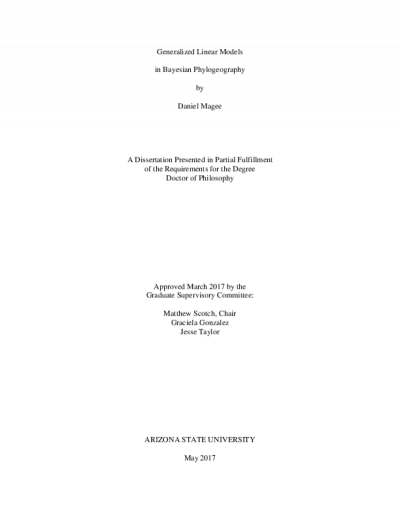
Description
Bayesian phylogeography is a framework that has enabled researchers to model the spatiotemporal diffusion of pathogens. In general, the framework assumes that discrete geographic sampling traits follow a continuous-time Markov chain process along the branches of an unknown phylogeny that is informed through nucleotide sequence data. Recently, this framework has been extended to model the transition rate matrix between discrete states as a generalized linear model (GLM) of predictors of interest to the pathogen. In this dissertation, I focus on these GLMs and describe their capabilities, limitations, and introduce a pipeline that may enable more researchers to utilize this framework.
I first demonstrate how a GLM can be employed and how the support for the predictors can be measured using influenza A/H5N1 in Egypt as an example. Secondly, I compare the GLM framework to two alternative frameworks of Bayesian phylogeography: one that uses an advanced computational technique and one that does not. For this assessment, I model the diffusion of influenza A/H3N2 in the United States during the 2014-15 flu season with five methods encapsulated by the three frameworks. I summarize metrics of the phylogenies created by each and demonstrate their reproducibility by performing analyses on several random sequence samples under a variety of population growth scenarios. Next, I demonstrate how discretization of the location trait for a given sequence set can influence phylogenies and support for predictors. That is, I perform several GLM analyses on a set of sequences and change how the sequences are pooled, then show how aggregating predictors at four levels of spatial resolution will alter posterior support. Finally, I provide a solution for researchers that wish to use the GLM framework but may be deterred by the tedious file-manipulation requirements that must be completed to do so. My pipeline, which is publicly available, should alleviate concerns pertaining to the difficulty and time-consuming nature of creating the files necessary to perform GLM analyses. This dissertation expands the knowledge of Bayesian phylogeographic GLMs and will facilitate the use of this framework, which may ultimately reveal the variables that drive the spread of pathogens.
I first demonstrate how a GLM can be employed and how the support for the predictors can be measured using influenza A/H5N1 in Egypt as an example. Secondly, I compare the GLM framework to two alternative frameworks of Bayesian phylogeography: one that uses an advanced computational technique and one that does not. For this assessment, I model the diffusion of influenza A/H3N2 in the United States during the 2014-15 flu season with five methods encapsulated by the three frameworks. I summarize metrics of the phylogenies created by each and demonstrate their reproducibility by performing analyses on several random sequence samples under a variety of population growth scenarios. Next, I demonstrate how discretization of the location trait for a given sequence set can influence phylogenies and support for predictors. That is, I perform several GLM analyses on a set of sequences and change how the sequences are pooled, then show how aggregating predictors at four levels of spatial resolution will alter posterior support. Finally, I provide a solution for researchers that wish to use the GLM framework but may be deterred by the tedious file-manipulation requirements that must be completed to do so. My pipeline, which is publicly available, should alleviate concerns pertaining to the difficulty and time-consuming nature of creating the files necessary to perform GLM analyses. This dissertation expands the knowledge of Bayesian phylogeographic GLMs and will facilitate the use of this framework, which may ultimately reveal the variables that drive the spread of pathogens.
Date Created
The date the item was original created (prior to any relationship with the ASU Digital Repositories.)
2017
Agent
- Author (aut): Magee, Daniel
- Thesis advisor (ths): Scotch, Matthew L
- Committee member: Gonzalez, Graciela H
- Committee member: Taylor, Jesse E
- Publisher (pbl): Arizona State University