Multiple imputation for two-level hierarchical models with categorical variables and missing at random data
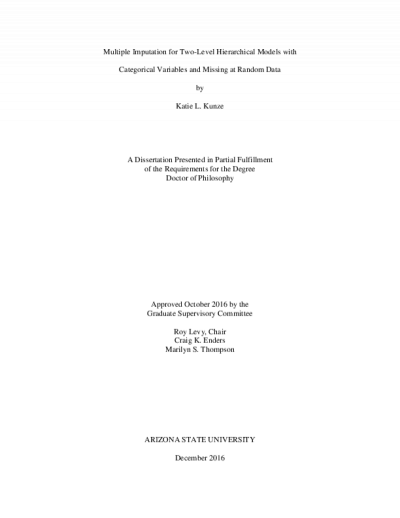
Description
Accurate data analysis and interpretation of results may be influenced by many potential factors. The factors of interest in the current work are the chosen analysis model(s), the presence of missing data, and the type(s) of data collected. If analysis models are used which a) do not accurately capture the structure of relationships in the data such as clustered/hierarchical data, b) do not allow or control for missing values present in the data, or c) do not accurately compensate for different data types such as categorical data, then the assumptions associated with the model have not been met and the results of the analysis may be inaccurate. In the presence of clustered
ested data, hierarchical linear modeling or multilevel modeling (MLM; Raudenbush & Bryk, 2002) has the ability to predict outcomes for each level of analysis and across multiple levels (accounting for relationships between levels) providing a significant advantage over single-level analyses. When multilevel data contain missingness, multilevel multiple imputation (MLMI) techniques may be used to model both the missingness and the clustered nature of the data. With categorical multilevel data with missingness, categorical MLMI must be used. Two such routines for MLMI with continuous and categorical data were explored with missing at random (MAR) data: a formal Bayesian imputation and analysis routine in JAGS (R/JAGS) and a common MLM procedure of imputation via Bayesian estimation in BLImP with frequentist analysis of the multilevel model in Mplus (BLImP/Mplus). Manipulated variables included interclass correlations, number of clusters, and the rate of missingness. Results showed that with continuous data, R/JAGS returned more accurate parameter estimates than BLImP/Mplus for almost all parameters of interest across levels of the manipulated variables. Both R/JAGS and BLImP/Mplus encountered convergence issues and returned inaccurate parameter estimates when imputing and analyzing dichotomous data. Follow-up studies showed that JAGS and BLImP returned similar imputed datasets but the choice of analysis software for MLM impacted the recovery of accurate parameter estimates. Implications of these findings and recommendations for further research will be discussed.
ested data, hierarchical linear modeling or multilevel modeling (MLM; Raudenbush & Bryk, 2002) has the ability to predict outcomes for each level of analysis and across multiple levels (accounting for relationships between levels) providing a significant advantage over single-level analyses. When multilevel data contain missingness, multilevel multiple imputation (MLMI) techniques may be used to model both the missingness and the clustered nature of the data. With categorical multilevel data with missingness, categorical MLMI must be used. Two such routines for MLMI with continuous and categorical data were explored with missing at random (MAR) data: a formal Bayesian imputation and analysis routine in JAGS (R/JAGS) and a common MLM procedure of imputation via Bayesian estimation in BLImP with frequentist analysis of the multilevel model in Mplus (BLImP/Mplus). Manipulated variables included interclass correlations, number of clusters, and the rate of missingness. Results showed that with continuous data, R/JAGS returned more accurate parameter estimates than BLImP/Mplus for almost all parameters of interest across levels of the manipulated variables. Both R/JAGS and BLImP/Mplus encountered convergence issues and returned inaccurate parameter estimates when imputing and analyzing dichotomous data. Follow-up studies showed that JAGS and BLImP returned similar imputed datasets but the choice of analysis software for MLM impacted the recovery of accurate parameter estimates. Implications of these findings and recommendations for further research will be discussed.
Date Created
The date the item was original created (prior to any relationship with the ASU Digital Repositories.)
2016
Agent
- Author (aut): Kunze, Katie L
- Thesis advisor (ths): Levy, Roy
- Committee member: Enders, Craig K.
- Committee member: Thompson, Marilyn S
- Publisher (pbl): Arizona State University