The Adaptive Lasso Procedure for Building a Traffic Forecasting Model
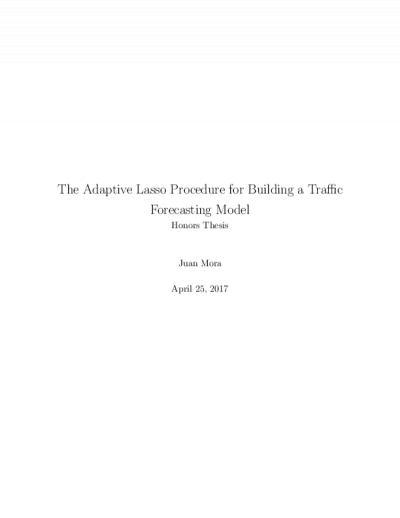
Description
This paper will begin by initially discussing the potential uses and challenges of efficient and accurate traffic forecasting. The data we used includes traffic volume from seven locations on a busy Athens street in April and May of 2000. This data was used as part of a traffic forecasting competition. Our initial observation, was that due to the volatility and oscillating nature of daily traffic volume, simple linear regression models will not perform well in predicting the time-series data. For this we present the Harmonic Time Series model. Such model (assuming all predictors are significant) will include a sinusoidal term for each time index within a period of data. Our assumption is that traffic volumes have a period of one week (which is evidenced by the graphs reproduced in our paper). This leads to a model that has 6,720 sine and cosine terms. This is clearly too many coefficients, so in an effort to avoid over-fitting and having an efficient model, we apply the sub-setting algorithm known as Adaptive Lass.
Date Created
The date the item was original created (prior to any relationship with the ASU Digital Repositories.)
2017-05
Agent
- Author (aut): Mora, Juan
- Thesis director: Kamarianakis, Ioannis
- Committee member: Yu, Wanchunzi
- Contributor (ctb): W. P. Carey School of Business
- Contributor (ctb): School of Mathematical and Statistical Sciences
- Contributor (ctb): Barrett, The Honors College