Properties of divergence-free kernel methods for approximation and solution of partial differential equations
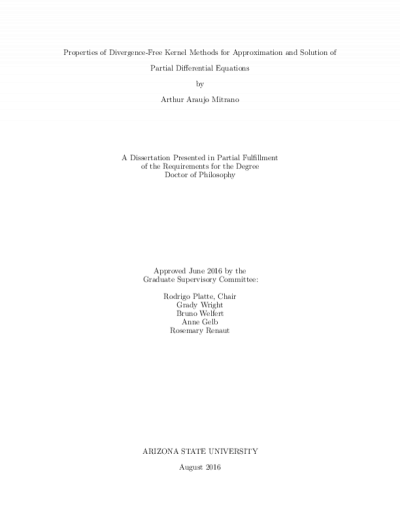
Description
Divergence-free vector field interpolants properties are explored on uniform and scattered nodes, and also their application to fluid flow problems. These interpolants may be applied to physical problems that require the approximant to have zero divergence, such as the velocity field in the incompressible Navier-Stokes equations and the magnetic and electric fields in the Maxwell's equations. In addition, the methods studied here are meshfree, and are suitable for problems defined on complex domains, where mesh generation is computationally expensive or inaccurate, or for problems where the data is only available at scattered locations.
The contributions of this work include a detailed comparison between standard and divergence-free radial basis approximations, a study of the Lebesgue constants for divergence-free approximations and their dependence on node placement, and an investigation of the flat limit of divergence-free interpolants. Finally, numerical solvers for the incompressible Navier-Stokes equations in primitive variables are implemented using discretizations based on traditional and divergence-free kernels. The numerical results are compared to reference solutions obtained with a spectral
method.
The contributions of this work include a detailed comparison between standard and divergence-free radial basis approximations, a study of the Lebesgue constants for divergence-free approximations and their dependence on node placement, and an investigation of the flat limit of divergence-free interpolants. Finally, numerical solvers for the incompressible Navier-Stokes equations in primitive variables are implemented using discretizations based on traditional and divergence-free kernels. The numerical results are compared to reference solutions obtained with a spectral
method.
Date Created
The date the item was original created (prior to any relationship with the ASU Digital Repositories.)
2016
Agent
- Author (aut): Araujo Mitrano, Arthur
- Thesis advisor (ths): Platte, Rodrigo
- Committee member: Wright, Grady
- Committee member: Welfert, Bruno
- Committee member: Gelb, Anne
- Committee member: Renaut, Rosemary
- Publisher (pbl): Arizona State University