Collaborative Thesis - Disrupting the Supply Chain: Application of A.I. and Machine Learning in Spares Inventory Management
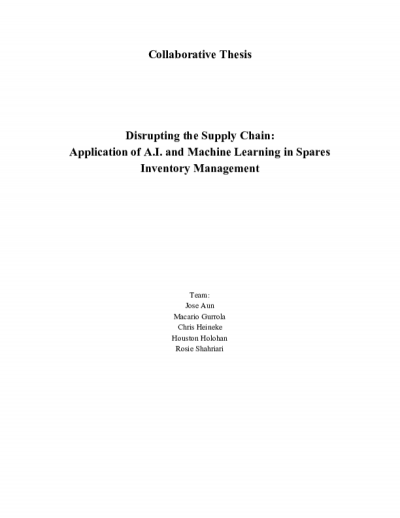
Description
This thesis discusses the case for Company X to improve its vast supply chain by implementing an artificial intelligence solution in the management of its spare parts inventory for manufacturing-related machinery. Currently, the company utilizes an inventory management system, based on previously set minimum and maximum thresholds, that doesn’t use predictive analytics to stock required spares inventory. This results in unnecessary costs and redundancies within the supply chain resulting in the stockout of spare parts required to repair machinery. Our research aimed to quantify the cost of these stockouts, and ultimately propose a solution to mitigate them. Through discussion with Company X, our findings led us to recommend the use of Artificial Intelligence (A.I.) within the inventory management system to better predict when stockouts would occur. As a result of data availability, our analysis began on a smaller scale, considering only a single manufacturing site at Company X. Later, our findings were extrapolated across all manufacturing sites. The analysis includes the cost of stockouts, the capital that would be saved with A.I. implementation, costs to implement this new A.I. software, and the final net present value (NPV) that Company X could expect in 10 years and 25 years. The NPV calculations explored two scenarios, an external partnership and the purchase of a small private company, that lead to our final recommendations regarding the implementation of an A.I. software solution in Company X’s spares inventory management system. Following the analysis, a qualitative discussion of the potential risks and market opportunities associated with the explored implementation scenarios further guided the determination of our final recommendations.
Date Created
The date the item was original created (prior to any relationship with the ASU Digital Repositories.)
2020-05
Agent
- Co-author: Holohan, Joseph Michael Houston
- Co-author: Shahriari, Rosie
- Co-author: Aun, Jose
- Co-author: Heineke, Christopher
- Co-author: Gurrola, Macario
- Thesis director: Simonson, Mark
- Committee member: Hertzel, Michael
- Contributor (ctb): Department of Finance
- Contributor (ctb): Barrett, The Honors College