Bayesian Phylogeography of Influenza A/H3N2 for the 2014-15 Season in the United States Using Three Frameworks of Ancestral State Reconstruction
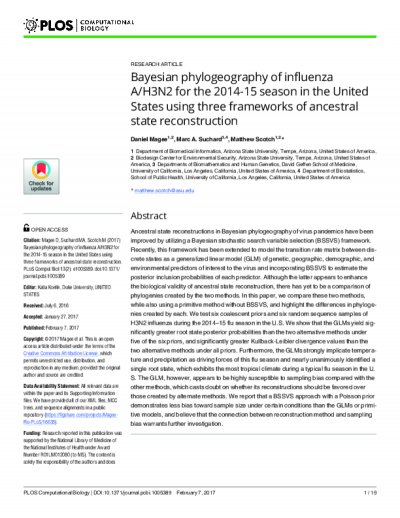
Ancestral state reconstructions in Bayesian phylogeography of virus pandemics have been improved by utilizing a Bayesian stochastic search variable selection (BSSVS) framework. Recently, this framework has been extended to model the transition rate matrix between discrete states as a generalized linear model (GLM) of genetic, geographic, demographic, and environmental predictors of interest to the virus and incorporating BSSVS to estimate the posterior inclusion probabilities of each predictor. Although the latter appears to enhance the biological validity of ancestral state reconstruction, there has yet to be a comparison of phylogenies created by the two methods.
In this paper, we compare these two methods, while also using a primitive method without BSSVS, and highlight the differences in phylogenies created by each. We test six coalescent priors and six random sequence samples of H3N2 influenza during the 2014–15 flu season in the U.S. We show that the GLMs yield significantly greater root state posterior probabilities than the two alternative methods under five of the six priors, and significantly greater Kullback-Leibler divergence values than the two alternative methods under all priors. Furthermore, the GLMs strongly implicate temperature and precipitation as driving forces of this flu season and nearly unanimously identified a single root state, which exhibits the most tropical climate during a typical flu season in the U.S.
The GLM, however, appears to be highly susceptible to sampling bias compared with the other methods, which casts doubt on whether its reconstructions should be favored over those created by alternate methods. We report that a BSSVS approach with a Poisson prior demonstrates less bias toward sample size under certain conditions than the GLMs or primitive models, and believe that the connection between reconstruction method and sampling bias warrants further investigation.
- Author (aut): Magee, Daniel
- Author (aut): Suchard, Marc A.
- Author (aut): Scotch, Matthew
- Contributor (ctb): College of Health Solutions