The Experimentation of Matrix for Product Emotion
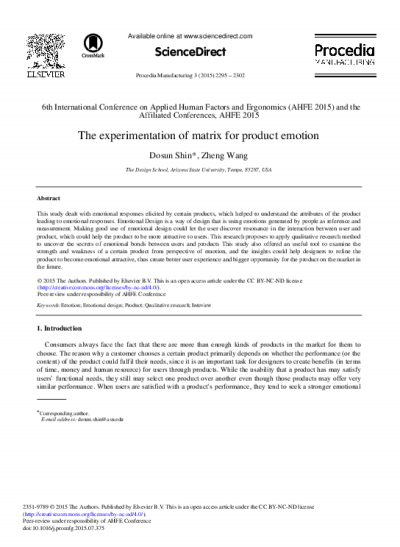
This study dealt with emotional responses elicited by certain products, which helped to understand the attributes of the product leading to emotional responses. Emotional Design is a way of design that is using emotions generated by people as reference and measurement. Making good use of emotional design could let the user discover resonance in the interaction between user and product, which could help the product to be more attractive to users. This research proposes to apply qualitative research method to uncover the secrets of emotional bonds between users and products This study also offered an useful tool to examine the strength and weakness of a certain product from perspective of emotion, and the insights could help designers to refine the product to become emotional attractive, thus create better user experience and bigger opportunity for the product on the market in the future.
- Author (aut): Shin, Dosun
- Author (aut): Wang, Zheng
- Contributor (ctb): Herberger Institute for Design and the Arts