Exploring Prompt-Based Methods for COVID-19 Misinformation Classification
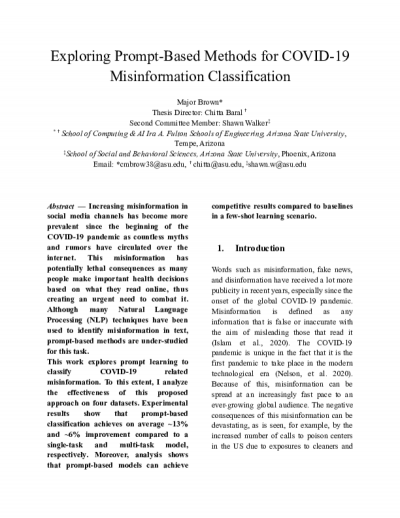
Description
Increasing misinformation in social media channels has become more prevalent since the beginning of the COVID-19 pandemic as countless myths and rumors have circulated over the internet. This misinformation has potentially lethal consequences as many people make important health decisions based on what they read online, thus creating an urgent need to combat it. Although many Natural Language Processing (NLP) techniques have been used to identify misinformation in text, prompt-based methods are under-studied for this task.
This work explores prompt learning to classify COVID-19 related misinformation. To this extent, I analyze the effectiveness of this proposed approach on four datasets. Experimental results show that prompt-based classification achieves on average ~13% and ~6% improvement compared to a single-task and multi-task model, respectively. Moreover, analysis shows that prompt-based models can achieve competitive results compared to baselines in a few-shot learning scenario.
Date Created
The date the item was original created (prior to any relationship with the ASU Digital Repositories.)
2022-05
Agent
- Author (aut): Brown, Clinton
- Thesis director: Baral, Chitta
- Committee member: Walker, Shawn
- Contributor (ctb): Barrett, The Honors College
- Contributor (ctb): School of International Letters and Cultures
- Contributor (ctb): Computer Science and Engineering Program