Recognizing Emotions: Developing a Linguistic Understanding of How Emotions Feed Into Speech Through Semantics and Facial Expressions
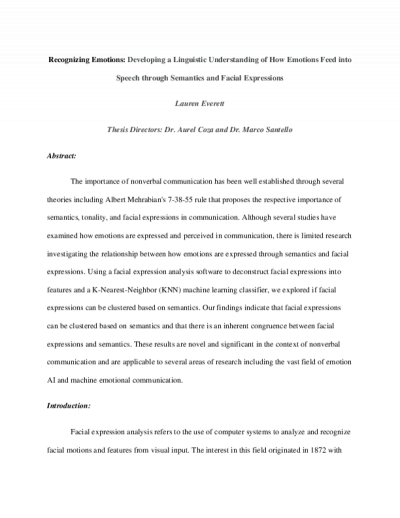
The importance of nonverbal communication has been well established through several theories including Albert Mehrabian's 7-38-55 rule that proposes the respective importance of semantics, tonality and facial expressions in communication. Although several studies have examined how emotions are expressed and preceived in communication, there is limited research investigating the relationship between how emotions are expressed through semantics and facial expressions. Using a facial expression analysis software to deconstruct facial expressions into features and a K-Nearest-Neighbor (KNN) machine learning classifier, we explored if facial expressions can be clustered based on semantics. Our findings indicate that facial expressions can be clustered based on semantics and that there is an inherent congruence between facial expressions and semantics. These results are novel and significant in the context of nonverbal communication and are applicable to several areas of research including the vast field of emotion AI and machine emotional communication.
- Author (aut): Everett, Lauren
- Thesis director: Coza, Aurel
- Committee member: Santello, Marco
- Contributor (ctb): Barrett, The Honors College
- Contributor (ctb): Harrington Bioengineering Program
- Contributor (ctb): Dean, W.P. Carey School of Business