Accurate and Efficient Autonomic Closure for Turbulent Flows
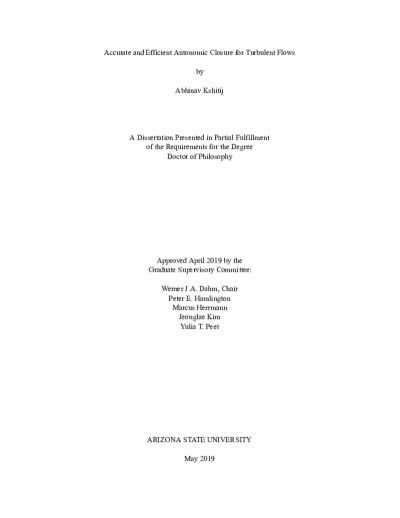
Description
Autonomic closure is a new general methodology for subgrid closures in large eddy simulations that circumvents the need to specify fixed closure models and instead allows a fully- adaptive self-optimizing closure. The closure is autonomic in the sense that the simulation itself determines the optimal relation at each point and time between any subgrid term and the variables in the simulation, through the solution of a local system identification problem. It is based on highly generalized representations of subgrid terms having degrees of freedom that are determined dynamically at each point and time in the simulation. This can be regarded as a very high-dimensional generalization of the dynamic approach used with some traditional prescribed closure models, or as a type of “data-driven” turbulence closure in which machine- learning methods are used with internal training data obtained at a test-filter scale at each point and time in the simulation to discover the local closure representation.
In this study, a priori tests were performed to develop accurate and efficient implementations of autonomic closure based on particular generalized representations and parameters associated with the local system identification of the turbulence state. These included the relative number of training points and bounding box size, which impact computational cost and generalizability of coefficients in the representation from the test scale to the LES scale. The focus was on studying impacts of these factors on the resulting accuracy and efficiency of autonomic closure for the subgrid stress. Particular attention was paid to the associated subgrid production field, including its structural features in which large forward and backward energy transfer are concentrated.
More than five orders of magnitude reduction in computational cost of autonomic closure was achieved in this study with essentially no loss of accuracy, primarily by using efficient frame-invariant forms for generalized representations that greatly reduce the number of degrees of freedom. The recommended form is a 28-coefficient representation that provides subgrid stress and production fields that are far more accurate in terms of structure and statistics than are traditional prescribed closure models.
In this study, a priori tests were performed to develop accurate and efficient implementations of autonomic closure based on particular generalized representations and parameters associated with the local system identification of the turbulence state. These included the relative number of training points and bounding box size, which impact computational cost and generalizability of coefficients in the representation from the test scale to the LES scale. The focus was on studying impacts of these factors on the resulting accuracy and efficiency of autonomic closure for the subgrid stress. Particular attention was paid to the associated subgrid production field, including its structural features in which large forward and backward energy transfer are concentrated.
More than five orders of magnitude reduction in computational cost of autonomic closure was achieved in this study with essentially no loss of accuracy, primarily by using efficient frame-invariant forms for generalized representations that greatly reduce the number of degrees of freedom. The recommended form is a 28-coefficient representation that provides subgrid stress and production fields that are far more accurate in terms of structure and statistics than are traditional prescribed closure models.
Date Created
The date the item was original created (prior to any relationship with the ASU Digital Repositories.)
2019
Agent
- Author (aut): Kshitij, Abhinav
- Thesis advisor (ths): Dahm, Werner J.A.
- Committee member: Herrmann, Marcus
- Committee member: Hamlington, Peter E
- Committee member: Peet, Yulia
- Committee member: Kim, Jeonglae
- Publisher (pbl): Arizona State University