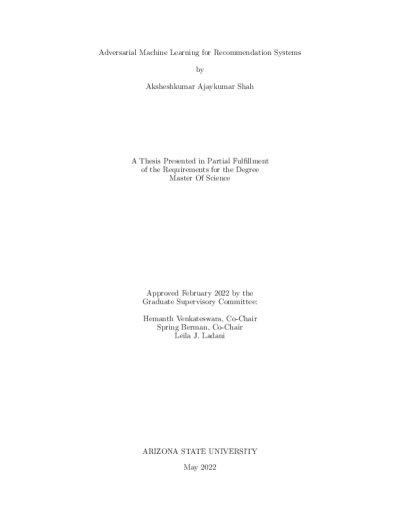
Description
Recently, Generative Adversarial Networks (GANs) have been applied to the problem of Cold-Start Recommendation, but the training performance of these models is hampered by the extreme sparsity in warm user purchase behavior. This thesis introduces a novel representation for user-vectors by combining user demographics and user preferences, making the model a hybrid system which uses Collaborative Filtering and Content Based Recommendation. This system models user purchase behavior using weighted user-product preferences (explicit feedback) rather than binary user-product interactions (implicit feedback). Using this a novel sparse adversarial model, Sparse ReguLarized Generative Adversarial Network (SRLGAN), is developed for Cold-Start Recommendation. SRLGAN leverages the sparse user-purchase behavior which ensures training stability and avoids over-fitting on warm users. The performance of SRLGAN is evaluated on two popular datasets and demonstrate state-of-the-art results.
Download count: 3
Details
Title
- Adversarial Machine Learning for Recommendation Systems
Contributors
- Shah, Aksheshkumar Ajaykumar (Author)
- Venkateswara, Hemanth (Thesis advisor)
- Berman, Spring (Thesis advisor)
- Ladani, Leila J (Committee member)
- Arizona State University (Publisher)
Date Created
The date the item was original created (prior to any relationship with the ASU Digital Repositories.)
2022
Subjects
Resource Type
Collections this item is in
Note
-
Partial requirement for: M.S., Arizona State University, 2022
-
Field of study: Mechanical Engineering