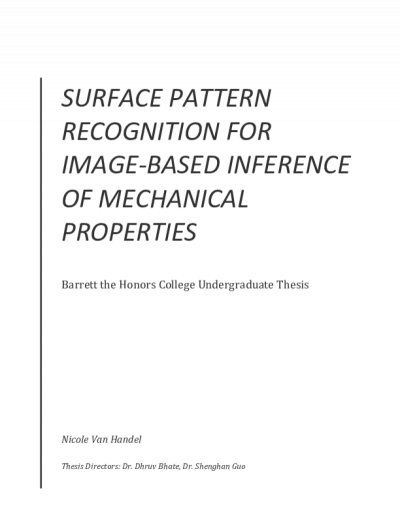
Description
A key aspect of understanding the behavior of materials and structures is the analysis of how they fail. A key aspect of failure analysis is the discipline of fractography, which identifies features of interest on fracture surfaces with the goal of revealing insights on the nature of defects and microstructure, and their interactions with the environment such as loading conditions. While fractography itself is a decades-old science, two aspects drive the need for this research: (i) Fractography remains a specialized domain of materials science where human subjectivity and experience play a large role in accurate determination of fracture modes and their relationship to the loading environment. (ii) Secondly, Additive Manufacturing (AM) is increasingly being used to create critical functional parts, where our understanding of failure mechanisms and how they relate to process and post-process conditions is nascent. Given these two challenges, this thesis conducted work to train convolutional neural network (CNN) models to analyze fracture surfaces in place of human experts and applies this to Inconel 718 specimens fabricated with the Laser Powder Bed Fusion (LPBF) process, as well as to traditional sheet metal specimens of the same alloy. This work intends to expand on previous work utilizing clustering methods through comparison of models developed using both manufacturing processes to demonstrate the effectiveness of the CNN approach, as well as elucidate insights into the nature of fracture modes in additively and traditionally manufactured thin-wall Inconel 718 specimens.
Details
Title
- Surface Pattern Recognition for Image-Based Inference of Mechanical Properties
Contributors
- Van Handel, Nicole (Author)
- Bhate, Dhruv (Thesis director, Committee member)
- Guo, Shenghan (Thesis director, Committee member)
- Barrett, The Honors College (Contributor)
- Engineering Programs (Contributor)
- Dean, W.P. Carey School of Business (Contributor)
- School of Mathematical and Statistical Sciences (Contributor)
Date Created
The date the item was original created (prior to any relationship with the ASU Digital Repositories.)
2022-05
Resource Type
Collections this item is in