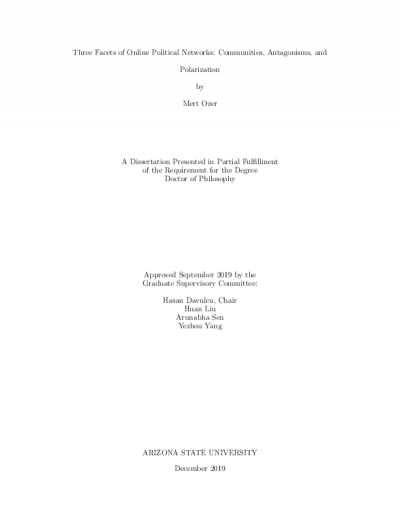
Description
Millions of users leave digital traces of their political engagements on social media platforms every day. Users form networks of interactions, produce textual content, like and share each others' content. This creates an invaluable opportunity to better understand the political engagements of internet users. In this proposal, I present three algorithmic solutions to three facets of online political networks; namely, detection of communities, antagonisms and the impact of certain types of accounts on political polarization. First, I develop a multi-view community detection algorithm to find politically pure communities. I find that word usage among other content types (i.e. hashtags, URLs) complement user interactions the best in accurately detecting communities.
Second, I focus on detecting negative linkages between politically motivated social media users. Major social media platforms do not facilitate their users with built-in negative interaction options. However, many political network analysis tasks rely on not only positive but also negative linkages. Here, I present the SocLSFact framework to detect negative linkages among social media users. It utilizes three pieces of information; sentiment cues of textual interactions, positive interactions, and socially balanced triads. I evaluate the contribution of each three aspects in negative link detection performance on multiple tasks.
Third, I propose an experimental setup that quantifies the polarization impact of automated accounts on Twitter retweet networks. I focus on a dataset of tragic Parkland shooting event and its aftermath. I show that when automated accounts are removed from the retweet network the network polarization decrease significantly, while a same number of accounts to the automated accounts are removed randomly the difference is not significant. I also find that prominent predictors of engagement of automatically generated content is not very different than what previous studies point out in general engaging content on social media. Last but not least, I identify accounts which self-disclose their automated nature in their profile by using expressions such as bot, chat-bot, or robot. I find that human engagement to self-disclosing accounts compared to non-disclosing automated accounts is much smaller. This observational finding can motivate further efforts into automated account detection research to prevent their unintended impact.
Second, I focus on detecting negative linkages between politically motivated social media users. Major social media platforms do not facilitate their users with built-in negative interaction options. However, many political network analysis tasks rely on not only positive but also negative linkages. Here, I present the SocLSFact framework to detect negative linkages among social media users. It utilizes three pieces of information; sentiment cues of textual interactions, positive interactions, and socially balanced triads. I evaluate the contribution of each three aspects in negative link detection performance on multiple tasks.
Third, I propose an experimental setup that quantifies the polarization impact of automated accounts on Twitter retweet networks. I focus on a dataset of tragic Parkland shooting event and its aftermath. I show that when automated accounts are removed from the retweet network the network polarization decrease significantly, while a same number of accounts to the automated accounts are removed randomly the difference is not significant. I also find that prominent predictors of engagement of automatically generated content is not very different than what previous studies point out in general engaging content on social media. Last but not least, I identify accounts which self-disclose their automated nature in their profile by using expressions such as bot, chat-bot, or robot. I find that human engagement to self-disclosing accounts compared to non-disclosing automated accounts is much smaller. This observational finding can motivate further efforts into automated account detection research to prevent their unintended impact.
Download count: 3
Details
Title
- Three Facets of Online Political Networks: Communities, Antagonisms, and Polarization
Contributors
- Ozer, Mert (Author)
- Davulcu, Hasan (Thesis advisor)
- Liu, Huan (Committee member)
- Sen, Arunabha (Committee member)
- Yang, Yezhou (Committee member)
- Arizona State University (Publisher)
Date Created
The date the item was original created (prior to any relationship with the ASU Digital Repositories.)
2019
Subjects
Resource Type
Collections this item is in
Note
-
Doctoral Dissertation Computer Science 2019