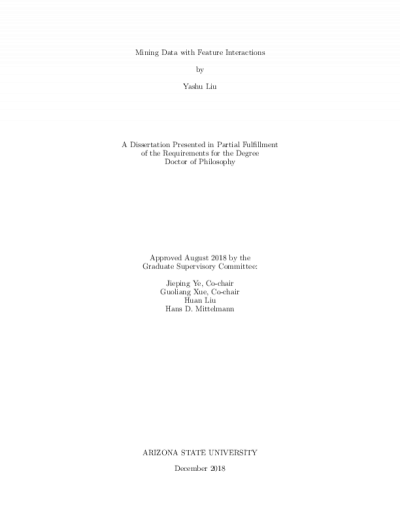
Description
Models using feature interactions have been applied successfully in many areas such as biomedical analysis, recommender systems. The popularity of using feature interactions mainly lies in (1) they are able to capture the nonlinearity of the data compared with linear effects and (2) they enjoy great interpretability. In this thesis, I propose a series of formulations using feature interactions for real world problems and develop efficient algorithms for solving them.
Specifically, I first propose to directly solve the non-convex formulation of the weak hierarchical Lasso which imposes weak hierarchy on individual features and interactions but can only be approximately solved by a convex relaxation in existing studies. I further propose to use the non-convex weak hierarchical Lasso formulation for hypothesis testing on the interaction features with hierarchical assumptions. Secondly, I propose a type of bi-linear models that take advantage of interactions of features for drug discovery problems where specific drug-drug pairs or drug-disease pairs are of interest. These models are learned by maximizing the number of positive data pairs that rank above the average score of unlabeled data pairs. Then I generalize the method to the case of using the top-ranked unlabeled data pairs for representative construction and derive an efficient algorithm for the extended formulation. Last but not least, motivated by a special form of bi-linear models, I propose a framework that enables simultaneously subgrouping data points and building specific models on the subgroups for learning on massive and heterogeneous datasets. Experiments on synthetic and real datasets are conducted to demonstrate the effectiveness or efficiency of the proposed methods.
Specifically, I first propose to directly solve the non-convex formulation of the weak hierarchical Lasso which imposes weak hierarchy on individual features and interactions but can only be approximately solved by a convex relaxation in existing studies. I further propose to use the non-convex weak hierarchical Lasso formulation for hypothesis testing on the interaction features with hierarchical assumptions. Secondly, I propose a type of bi-linear models that take advantage of interactions of features for drug discovery problems where specific drug-drug pairs or drug-disease pairs are of interest. These models are learned by maximizing the number of positive data pairs that rank above the average score of unlabeled data pairs. Then I generalize the method to the case of using the top-ranked unlabeled data pairs for representative construction and derive an efficient algorithm for the extended formulation. Last but not least, motivated by a special form of bi-linear models, I propose a framework that enables simultaneously subgrouping data points and building specific models on the subgroups for learning on massive and heterogeneous datasets. Experiments on synthetic and real datasets are conducted to demonstrate the effectiveness or efficiency of the proposed methods.
Details
Title
- Mining Data with Feature Interactions
Contributors
- Liu, Yashu (Author)
- Ye, Jieping (Thesis advisor)
- Xue, Guoliang (Thesis advisor)
- Liu, Huan (Committee member)
- Mittelmann, Hans D (Committee member)
- Arizona State University (Publisher)
Date Created
The date the item was original created (prior to any relationship with the ASU Digital Repositories.)
2018
Resource Type
Collections this item is in
Note
-
Doctoral Dissertation Computer Science 2018