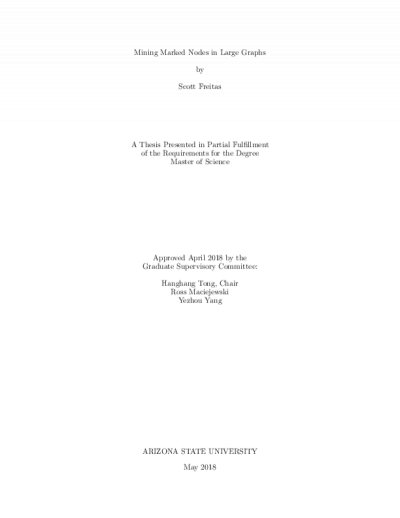
Description
With the rise of the Big Data Era, an exponential amount of network data is being generated at an unprecedented rate across a wide-range of high impact micro and macro areas of research---from protein interaction to social networks. The critical challenge is translating this large scale network data into actionable information.
A key task in the data translation is the analysis of network connectivity via marked nodes---the primary focus of our research. We have developed a framework for analyzing network connectivity via marked nodes in large scale graphs, utilizing novel algorithms in three interrelated areas: (1) analysis of a single seed node via it’s ego-centric network (AttriPart algorithm); (2) pathway identification between two seed nodes (K-Simple Shortest Paths Multithreaded and Search Reduced (KSSPR) algorithm); and (3) tree detection, defining the interaction between three or more seed nodes (Shortest Path MST algorithm).
In an effort to address both fundamental and applied research issues, we have developed the LocalForcasting algorithm to explore how network connectivity analysis can be applied to local community evolution and recommender systems. The goal is to apply the LocalForecasting algorithm to various domains---e.g., friend suggestions in social networks or future collaboration in co-authorship networks. This algorithm utilizes link prediction in combination with the AttriPart algorithm to predict future connections in local graph partitions.
Results show that our proposed AttriPart algorithm finds up to 1.6x denser local partitions, while running approximately 43x faster than traditional local partitioning techniques (PageRank-Nibble). In addition, our LocalForecasting algorithm demonstrates a significant improvement in the number of nodes and edges correctly predicted over baseline methods. Furthermore, results for the KSSPR algorithm demonstrate a speed-up of up to 2.5x the standard k-simple shortest paths algorithm.
A key task in the data translation is the analysis of network connectivity via marked nodes---the primary focus of our research. We have developed a framework for analyzing network connectivity via marked nodes in large scale graphs, utilizing novel algorithms in three interrelated areas: (1) analysis of a single seed node via it’s ego-centric network (AttriPart algorithm); (2) pathway identification between two seed nodes (K-Simple Shortest Paths Multithreaded and Search Reduced (KSSPR) algorithm); and (3) tree detection, defining the interaction between three or more seed nodes (Shortest Path MST algorithm).
In an effort to address both fundamental and applied research issues, we have developed the LocalForcasting algorithm to explore how network connectivity analysis can be applied to local community evolution and recommender systems. The goal is to apply the LocalForecasting algorithm to various domains---e.g., friend suggestions in social networks or future collaboration in co-authorship networks. This algorithm utilizes link prediction in combination with the AttriPart algorithm to predict future connections in local graph partitions.
Results show that our proposed AttriPart algorithm finds up to 1.6x denser local partitions, while running approximately 43x faster than traditional local partitioning techniques (PageRank-Nibble). In addition, our LocalForecasting algorithm demonstrates a significant improvement in the number of nodes and edges correctly predicted over baseline methods. Furthermore, results for the KSSPR algorithm demonstrate a speed-up of up to 2.5x the standard k-simple shortest paths algorithm.
Details
Title
- Mining Marked Nodes in Large Graphs
Contributors
- Freitas, Scott (Author)
- Tong, Hanghang (Thesis advisor)
- Maciejewski, Ross (Committee member)
- Yang, Yezhou (Committee member)
- Arizona State University (Publisher)
Date Created
The date the item was original created (prior to any relationship with the ASU Digital Repositories.)
2018
Subjects
Resource Type
Collections this item is in
Note
-
Masters Thesis Computer Science 2018