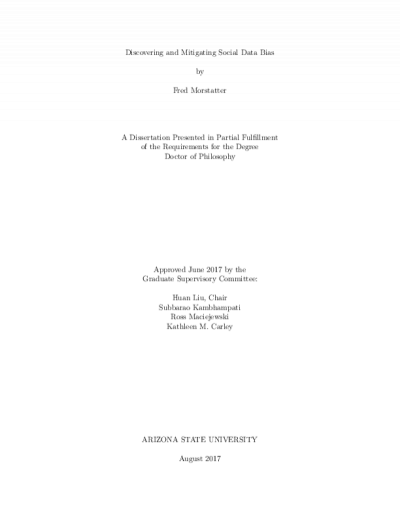
Description
Exabytes of data are created online every day. This deluge of data is no more apparent than it is on social media. Naturally, finding ways to leverage this unprecedented source of human information is an active area of research. Social media platforms have become laboratories for conducting experiments about people at scales thought unimaginable only a few years ago.
Researchers and practitioners use social media to extract actionable patterns such as where aid should be distributed in a crisis. However, the validity of these patterns relies on having a representative dataset. As this dissertation shows, the data collected from social media is seldom representative of the activity of the site itself, and less so of human activity. This means that the results of many studies are limited by the quality of data they collect.
The finding that social media data is biased inspires the main challenge addressed by this thesis. I introduce three sets of methodologies to correct for bias. First, I design methods to deal with data collection bias. I offer a methodology which can find bias within a social media dataset. This methodology works by comparing the collected data with other sources to find bias in a stream. The dissertation also outlines a data collection strategy which minimizes the amount of bias that will appear in a given dataset. It introduces a crawling strategy which mitigates the amount of bias in the resulting dataset. Second, I introduce a methodology to identify bots and shills within a social media dataset. This directly addresses the concern that the users of a social media site are not representative. Applying these methodologies allows the population under study on a social media site to better match that of the real world. Finally, the dissertation discusses perceptual biases, explains how they affect analysis, and introduces computational approaches to mitigate them.
The results of the dissertation allow for the discovery and removal of different levels of bias within a social media dataset. This has important implications for social media mining, namely that the behavioral patterns and insights extracted from social media will be more representative of the populations under study.
Researchers and practitioners use social media to extract actionable patterns such as where aid should be distributed in a crisis. However, the validity of these patterns relies on having a representative dataset. As this dissertation shows, the data collected from social media is seldom representative of the activity of the site itself, and less so of human activity. This means that the results of many studies are limited by the quality of data they collect.
The finding that social media data is biased inspires the main challenge addressed by this thesis. I introduce three sets of methodologies to correct for bias. First, I design methods to deal with data collection bias. I offer a methodology which can find bias within a social media dataset. This methodology works by comparing the collected data with other sources to find bias in a stream. The dissertation also outlines a data collection strategy which minimizes the amount of bias that will appear in a given dataset. It introduces a crawling strategy which mitigates the amount of bias in the resulting dataset. Second, I introduce a methodology to identify bots and shills within a social media dataset. This directly addresses the concern that the users of a social media site are not representative. Applying these methodologies allows the population under study on a social media site to better match that of the real world. Finally, the dissertation discusses perceptual biases, explains how they affect analysis, and introduces computational approaches to mitigate them.
The results of the dissertation allow for the discovery and removal of different levels of bias within a social media dataset. This has important implications for social media mining, namely that the behavioral patterns and insights extracted from social media will be more representative of the populations under study.
Download count: 3
Details
Title
- Discovering and Mitigating Social Data Bias
Contributors
- Morstatter, Fred (Author)
- Liu, Huan (Thesis advisor)
- Kambhampati, Subbarao (Committee member)
- Maciejewski, Ross (Committee member)
- Carley, Kathleen M. (Committee member)
- Arizona State University (Publisher)
Date Created
The date the item was original created (prior to any relationship with the ASU Digital Repositories.)
2017
Subjects
Resource Type
Collections this item is in
Note
-
Doctoral Dissertation Computer Science 2017