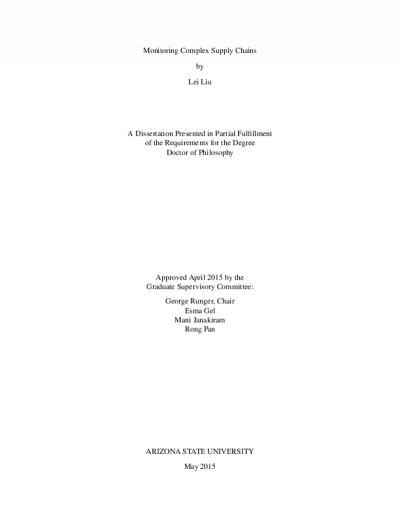
Description
The complexity of supply chains (SC) has grown rapidly in recent years, resulting in an increased difficulty to evaluate and visualize performance. Consequently, analytical approaches to evaluate SC performance in near real time relative to targets and plans are important to detect and react to deviations in order to prevent major disruptions.
Manufacturing anomalies, inaccurate forecasts, and other problems can lead to SC disruptions. Traditional monitoring methods are not sufficient in this respect, because com- plex SCs feature changes in manufacturing tasks (dynamic complexity) and carry a large number of stock keeping units (detail complexity). Problems are easily confounded with normal system variations.
Motivated by these real challenges faced by modern SC, new surveillance solutions are proposed to detect system deviations that could lead to disruptions in a complex SC. To address supply-side deviations, the fitness of different statistics that can be extracted from the enterprise resource planning system is evaluated. A monitoring strategy is first proposed for SCs featuring high levels of dynamic complexity. This presents an opportunity for monitoring methods to be applied in a new, rich domain of SC management. Then a monitoring strategy, called Heat Map Contrasts (HMC), which converts monitoring into a series of classification problems, is used to monitor SCs with both high levels of dynamic and detail complexities. Data from a semiconductor SC simulator are used to compare the methods with other alternatives under various failure cases, and the results illustrate the viability of our methods.
To address demand-side deviations, a new method of quantifying forecast uncer- tainties using the progression of forecast updates is presented. It is illustrated that a rich amount of information is available in rolling horizon forecasts. Two proactive indicators of future forecast errors are extracted from the forecast stream. This quantitative method re- quires no knowledge of the forecasting model itself and has shown promising results when applied to two datasets consisting of real forecast updates.
Manufacturing anomalies, inaccurate forecasts, and other problems can lead to SC disruptions. Traditional monitoring methods are not sufficient in this respect, because com- plex SCs feature changes in manufacturing tasks (dynamic complexity) and carry a large number of stock keeping units (detail complexity). Problems are easily confounded with normal system variations.
Motivated by these real challenges faced by modern SC, new surveillance solutions are proposed to detect system deviations that could lead to disruptions in a complex SC. To address supply-side deviations, the fitness of different statistics that can be extracted from the enterprise resource planning system is evaluated. A monitoring strategy is first proposed for SCs featuring high levels of dynamic complexity. This presents an opportunity for monitoring methods to be applied in a new, rich domain of SC management. Then a monitoring strategy, called Heat Map Contrasts (HMC), which converts monitoring into a series of classification problems, is used to monitor SCs with both high levels of dynamic and detail complexities. Data from a semiconductor SC simulator are used to compare the methods with other alternatives under various failure cases, and the results illustrate the viability of our methods.
To address demand-side deviations, a new method of quantifying forecast uncer- tainties using the progression of forecast updates is presented. It is illustrated that a rich amount of information is available in rolling horizon forecasts. Two proactive indicators of future forecast errors are extracted from the forecast stream. This quantitative method re- quires no knowledge of the forecasting model itself and has shown promising results when applied to two datasets consisting of real forecast updates.
Details
Title
- Monitoring complex supply chains
Contributors
- Liu, Lei (Author)
- Runger, George C. (Thesis advisor)
- Gel, Esma (Committee member)
- Pan, Rong (Committee member)
- Janakiram, Mani (Committee member)
- Arizona State University (Publisher)
Date Created
The date the item was original created (prior to any relationship with the ASU Digital Repositories.)
2015
Subjects
Resource Type
Collections this item is in
Note
-
thesisPartial requirement for: Ph.D., Arizona State University, 2015
-
bibliographyIncludes bibliographical references (pages 101-106)
-
Field of study: Industrial engineering
Citation and reuse
Statement of Responsibility
by Lei Liu