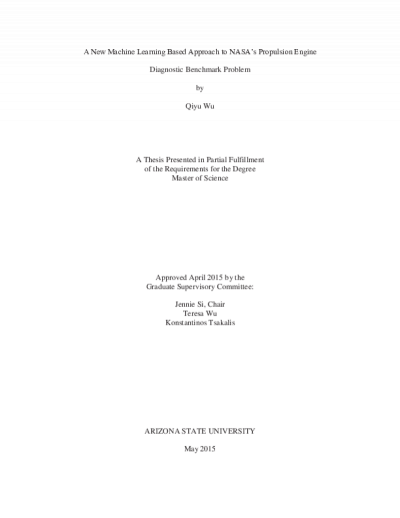
Description
Gas turbine engine for aircraft propulsion represents one of the most physics-complex and safety-critical systems in the world. Its failure diagnostic is challenging due to the complexity of the model system, difficulty involved in practical testing and the infeasibility of creating homogeneous diagnostic performance evaluation criteria for the diverse engine makes.
NASA has designed and publicized a standard benchmark problem for propulsion engine gas path diagnostic that enables comparisons among different engine diagnostic approaches. Some traditional model-based approaches and novel purely data-driven approaches such as machine learning, have been applied to this problem.
This study focuses on a different machine learning approach to the diagnostic problem. Some most common machine learning techniques, such as support vector machine, multi-layer perceptron, and self-organizing map are used to help gain insight into the different engine failure modes from the perspective of big data. They are organically integrated to achieve good performance based on a good understanding of the complex dataset.
The study presents a new hierarchical machine learning structure to enhance classification accuracy in NASA's engine diagnostic benchmark problem. The designed hierarchical structure produces an average diagnostic accuracy of 73.6%, which outperforms comparable studies that were most recently published.
NASA has designed and publicized a standard benchmark problem for propulsion engine gas path diagnostic that enables comparisons among different engine diagnostic approaches. Some traditional model-based approaches and novel purely data-driven approaches such as machine learning, have been applied to this problem.
This study focuses on a different machine learning approach to the diagnostic problem. Some most common machine learning techniques, such as support vector machine, multi-layer perceptron, and self-organizing map are used to help gain insight into the different engine failure modes from the perspective of big data. They are organically integrated to achieve good performance based on a good understanding of the complex dataset.
The study presents a new hierarchical machine learning structure to enhance classification accuracy in NASA's engine diagnostic benchmark problem. The designed hierarchical structure produces an average diagnostic accuracy of 73.6%, which outperforms comparable studies that were most recently published.
Download count: 4
Details
Title
- A new machine learning based approach to NASA's propulsion engine diagnostic benchmark problem
Contributors
- Wu, Qiyu (Author)
- Si, Jennie (Thesis advisor)
- Wu, Teresa (Committee member)
- Tsakalis, Konstantinos (Committee member)
- Arizona State University (Publisher)
Date Created
The date the item was original created (prior to any relationship with the ASU Digital Repositories.)
2015
Subjects
Resource Type
Collections this item is in
Note
-
thesisPartial requirement for: M.S., Arizona State University, 2015
-
bibliographyIncludes bibliographical references (pages 28-29)
-
Field of study: Electrical engineering
Citation and reuse
Statement of Responsibility
by Qiyu Wu