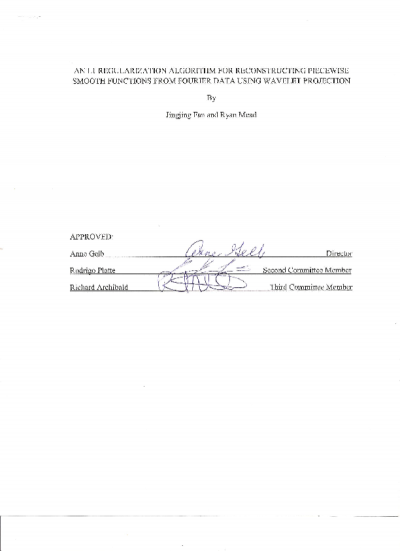
Description
Imaging technologies such as Magnetic Resonance Imaging (MRI) and Synthetic Aperture Radar (SAR) collect Fourier data and then process the data to form images. Because images are piecewise smooth, the Fourier partial sum (i.e. direct inversion of the Fourier data) yields a poor approximation, with spurious oscillations forming at the interior edges of the image and reduced accuracy overall. This is the well known Gibbs phenomenon and many attempts have been made to rectify its effects. Previous algorithms exploited the sparsity of edges in the underlying image as a constraint with which to optimize for a solution with reduced spurious oscillations. While the sparsity enforcing algorithms are fairly effective, they are sensitive to several issues, including undersampling and noise. Because of the piecewise nature of the underlying image, we theorize that projecting the solution onto the wavelet basis would increase the overall accuracy. Thus in this investigation we develop an algorithm that continues to exploit the sparsity of edges in the underlying image while also seeking to represent the solution using the wavelet rather than Fourier basis. Our method successfully decreases the effect of the Gibbs phenomenon and provides a good approximation for the underlying image. The primary advantages of our method is its robustness to undersampling and perturbations in the optimization parameters.
Details
Title
- An l1 Regularization Algorithm for Reconstructing Piecewise Smooth Functions from Fourier Data Using Wavelet Projection
Contributors
- Fan, Jingjing (Co-author)
- Mead, Ryan (Co-author)
- Gelb, Anne (Thesis director)
- Platte, Rodrigo (Committee member)
- Archibald, Richard (Committee member)
- School of Music (Contributor)
- School of Mathematical and Statistical Sciences (Contributor)
- Barrett, The Honors College (Contributor)
Date Created
The date the item was original created (prior to any relationship with the ASU Digital Repositories.)
2015-12
Resource Type
Collections this item is in