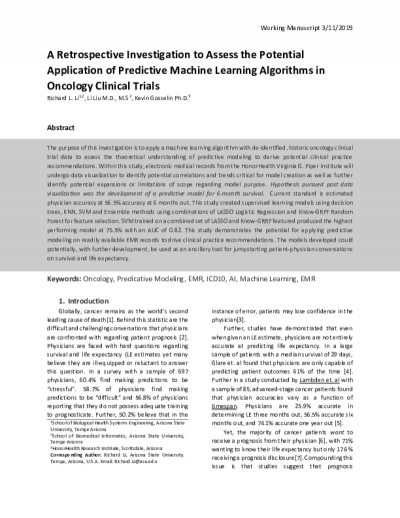
Description
The purpose of this investigation is to apply a machine learning algorithm with de-identified, historic oncology clinical trial data to assess the theoretical understanding of predictive modeling to derive potential clinical practice recommendations. Within this study, electronic medical records from the HonorHealth Virginia G. Piper Institute will undergo data visualization to identify potential correlations and trends critical for model creation as well as further identify potential expansions or limitations of scope regarding model purpose. Hypothesis pursued post data visualization was the development of a predictive model for 6-month survival. Current standard is estimated physician accuracy at 56.5% accuracy at 6 months out. This study created supervised learning models using decision trees, KNN, SVM and Ensemble methods using combinations of LASSO Logistic Regression and Know-GRFF Random Forest for feature selection. SVM trained on a combined set of LASSO and Know-GRRF featured produced the highest performing model at 75.5% with an AUC of 0.82. This study demonstrates the potential for applying predictive modeling on readily available EMR records to drive clinical practice recommendations. The models developed could potentially, with further development, be used as an ancillary tool for jumpstarting patient-physician conversations on survival and life expectancy.
Details
Title
- A Retrospective Investigation to Assess the Potential Application of Predictive Machine Learning Algorithms in Oncology Clinical Trials
Contributors
- Li, Richard Longfei (Co-author)
- Liu, Li (Co-author, Thesis director)
- Gosselin, Kevin (Co-author, Committee member)
- Harrington Bioengineering Program (Contributor, Contributor)
- Barrett, The Honors College (Contributor)
Date Created
The date the item was original created (prior to any relationship with the ASU Digital Repositories.)
2019-05
Resource Type
Collections this item is in