Moving Target Defense: Defending against Adversarial Defense
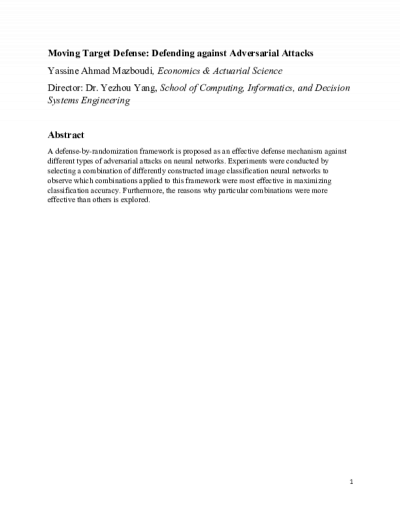
Description
A defense-by-randomization framework is proposed as an effective defense mechanism against different types of adversarial attacks on neural networks. Experiments were conducted by selecting a combination of differently constructed image classification neural networks to observe which combinations applied to this framework were most effective in maximizing classification accuracy. Furthermore, the reasons why particular combinations were more effective than others is explored.
Date Created
The date the item was original created (prior to any relationship with the ASU Digital Repositories.)
2019-05
Agent
- Author (aut): Mazboudi, Yassine Ahmad
- Thesis director: Yang, Yezhou
- Committee member: Ren, Yi
- Contributor (ctb): School of Mathematical and Statistical Sciences
- Contributor (ctb): Economics Program in CLAS
- Contributor (ctb): Barrett, The Honors College