Gram-ART Applied to Music Recommendation Services
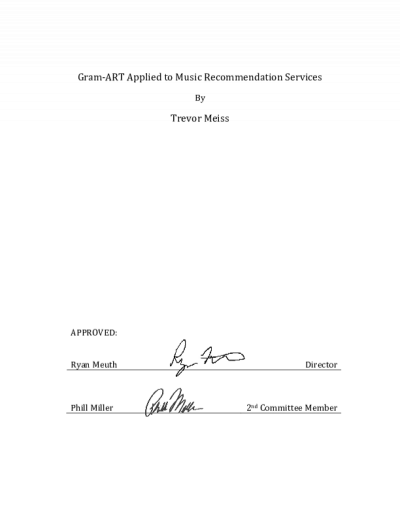
Description
In this paper we explore the design, implementation, and analysis of two different approaches for providing music recommendations to targeted users by implementing the Gram-ART unsupervised learning algorithm. We provide a content filtering approach using a dataset of one million songs which include various metadata tags and a collaborative filtering approach using the listening histories of over one million users. The two methods are evaluated by their results from Million Song Dataset Challenge. While both placed near the top third of the 150 challenge participants, the knowledge gained from the experiments will help further refine the process and likely produced much higher results in a system with the potential to scale several magnitudes.
Date Created
The date the item was original created (prior to any relationship with the ASU Digital Repositories.)
2015-05
Agent
- Author (aut): Meiss, Trevor
- Thesis director: Meuth, Ryan
- Committee member: Miller, Phill
- Contributor (ctb): Barrett, The Honors College