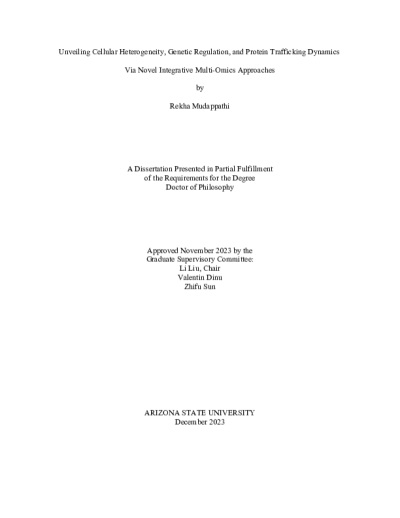
Description
Advancements in high-throughput biotechnologies have generated large-scale multi-omics datasets encompassing diverse dimensions such as genomics, epigenomics, transcriptomics, proteomics, metabolomics, metagenomics, and phenomics. Traditionally, statistical and machine learning-based approaches utilize single-omics data sources to uncover molecular signatures, dissect complicated cellular mechanisms, and predict clinical results. However, to capture the multifaceted pathological mechanisms, integrative multi-omics analysis is needed that can provide a comprehensive picture of the disease. Here, I present three novel approaches to multi-omics integrative analysis. I introduce a single-cell integrative clustering method, which leverages multi-omics to enhance the resolution of cell subpopulations. Applied to a Cellular Indexing of Transcriptomes and Epitopes (CITE-Seq) dataset from human Acute Myeloid Lymphoma (AML) and control samples, this approach unveiled nuanced cell populations that otherwise remain elusive. I then shift the focus to a computational framework to discover transcriptional regulatory trios in which a transcription factor binds to a regulatory element harboring a genetic variant and subsequently differentially regulates the transcription level of a target gene. Applied to whole-exome, whole-genome, and transcriptome data of multiple myeloma samples, this approach discovered synergetic cis-acting and trans-acting regulatory elements associated with tumorigenesis. The next part of this work introduces a novel methodology that leverages the transcriptome and surface protein data at the single-cell level produced by CITE-Seq to model the intracellular protein trafficking process. Applied to COVID-19 samples, this approach revealed dysregulated protein trafficking associated with the severity of the infection.
Download count: 8
Details
Title
- Unveiling Cellular Heterogeneity, Genetic Regulation, and Protein Trafficking Dynamics Via Novel Integrative Multi-Omic Approaches
Contributors
- Mudappathi, Rekha (Author)
- Liu, Li (Thesis advisor)
- Dinu, Valentin (Committee member)
- Sun, Zhifu (Committee member)
- Arizona State University (Publisher)
Date Created
The date the item was original created (prior to any relationship with the ASU Digital Repositories.)
2023
Subjects
Resource Type
Collections this item is in
Note
-
Partial requirement for: Ph.D., Arizona State University, 2023
-
Field of study: Biomedical Informatics