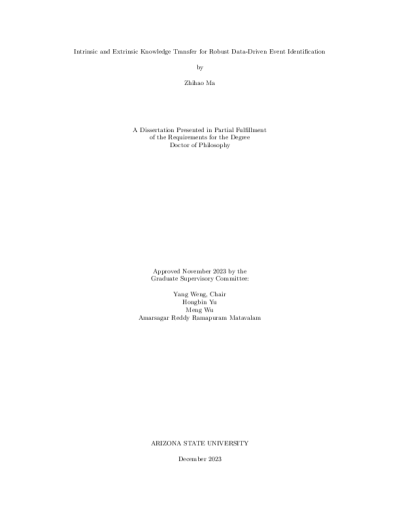
Description
The integration of Distributed Energy Resources (DER), including wind energy and photovoltaic (PV) panels, into power systems, increases the potential for events that could lead to outages and cascading failures. This risk is heightened by the limited dynamic information in energy grid datasets, primarily due to sparse Phasor Measurement Units (PMUs) placement. This data quality issue underscores the need for effective methodologies to manage these challenges. One significant challenge is the data gaps in low-resolution (LR) data from RTU and smart meters, hindering robust machine learning (ML) applications. To address this, a systematic approach involves preparing data effectively and designing efficient event detection methods, utilizing both intrinsic physics and extrinsic correlations from power systems. The process begins by interpolating LR data using high-resolution (HR) data, aiming to create virtual PMUs for improved grid management. Current interpolation methods often overlook extrinsic spatial-temporal correlations and intrinsic governing equations like Ordinary Differential Equations (ODEs) or Differential Algebraic Equations (DAEs). Physics-Informed Neural Networks (PINNs) are used for this purpose, though they face challenges with limited LR samples. The solution involves exploring the embedding space governed by ODEs/DAEs, generating extrinsic correlations for initial LR data imputation, and enforcing intrinsic physical constraints for refinement. After data preparation, event data dimensions such as spatial, temporal, and measurement categories are recovered in a tensor. To prevent overfitting, common in traditional ML methods, tensor decomposition is used. This technique merges intrinsic and physical information across dimensions, yielding informative and compact feature vectors for efficient feature extraction and learning in event detection. Lastly, in grids with insufficient data, knowledge transfer from grids with similar event patterns is a viable solution. This involves optimizing projected and transferred vectors from tensor decomposition to maximize common knowledge utilization across grids. This strategy identifies common features, enhancing the robustness and efficiency of ML event detection models, even in scenarios with limited event data.
Details
Title
- Intrinsic and Extrinsic Knowledge Transfer for Robust Data-Driven Event Identification
Contributors
- Ma, Zhihao (Author)
- Weng, Yang (Thesis advisor)
- Wu, Meng (Committee member)
- Yu, Hongbin (Committee member)
- Matavalam, Amarsagar Reddy Ramapuram (Committee member)
- Arizona State University (Publisher)
Date Created
The date the item was original created (prior to any relationship with the ASU Digital Repositories.)
2023
Subjects
Resource Type
Collections this item is in
Note
- Partial requirement for: Ph.D., Arizona State University, 2023
- Field of study: Electrical Engineering