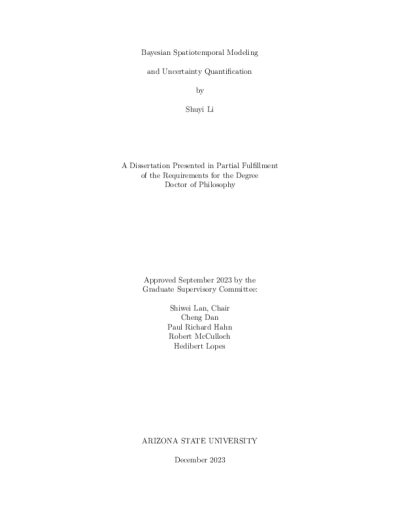
Description
Uncertainty Quantification (UQ) is crucial in assessing the reliability of predictivemodels that make decisions for human experts in a data-rich world. The Bayesian approach to UQ for inverse problems has gained popularity. However, addressing UQ in high-dimensional inverse problems is challenging due to the intensity and inefficiency of Markov Chain Monte Carlo (MCMC) based Bayesian inference methods. Consequently, the first primary focus of this thesis is enhancing efficiency and scalability for UQ in inverse problems.
On the other hand, the omnipresence of spatiotemporal data, particularly in areas like traffic analysis, underscores the need for effectively addressing inverse problems with spatiotemporal observations. Conventional solutions often overlook spatial or temporal correlations, resulting in underutilization of spatiotemporal interactions for parameter learning. Appropriately modeling spatiotemporal observations in inverse problems thus forms another pivotal research avenue.
In terms of UQ methodologies, the calibration-emulation-sampling (CES) scheme has emerged as effective for large-dimensional problems. I introduce a novel CES approach by employing deep neural network (DNN) models during the emulation and sampling phase. This approach not only enhances computational efficiency but also diminishes sensitivity to training set variations. The newly devised “Dimension- Reduced Emulative Autoencoder Monte Carlo (DREAM)” algorithm scales Bayesian UQ up to thousands of dimensions in physics-constrained inverse problems. The algorithm’s effectiveness is exemplified through elliptic and advection-diffusion inverse problems.
In the realm of spatiotemporal modeling, I propose to use Spatiotemporal Gaussian processes (STGP) in likelihood modeling and Spatiotemporal Besov processes (STBP) in prior modeling separately. These approaches highlight the efficacy of incorporat- ing spatial and temporal information for enhanced parameter estimation and UQ. Additionally, the superiority of STGP is demonstrated compared to static and time- averaged methods in time-dependent advection-diffusion partial differential equation
(PDE) and three chaotic ordinary differential equations (ODE). Expanding upon Besov Process (BP), a method known for sparsity-promotion and edge-preservation, STBP is introduced to capture spatial data features and model temporal correlations by replacing the random coefficients in the series expansion with stochastic time functions following Q-exponential process(Q-EP). This advantage is showcased in dynamic computerized tomography (CT) reconstructions through comparison with classic STGP and a time-uncorrelated approach.
Details
Title
- Bayesian Spatiotemporal Modeling and Uncertainty Quantification
Contributors
- Li, Shuyi (Author)
- Lan, Shiwei (Thesis advisor)
- Hahn, Paul (Committee member)
- McCulloch, Robert (Committee member)
- Dan, Cheng (Committee member)
- Lopes, Hedibert (Committee member)
- Arizona State University (Publisher)
Date Created
The date the item was original created (prior to any relationship with the ASU Digital Repositories.)
2023
Subjects
Resource Type
Collections this item is in
Note
- Partial requirement for: Ph.D., Arizona State University, 2023
- Field of study: Statistics