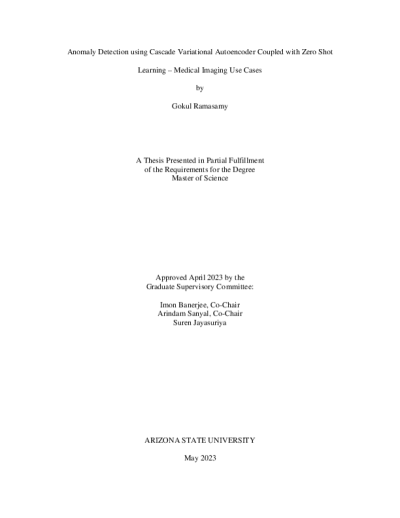
Description
Detection of anomalies before they are included in the downstream diagnosis/prognosis models is an important criterion for maintaining the medical AI imaging model performance across internal and external datasets. Furthermore, the need to curate huge amounts of data to train supervised models that produce precise results also requires an automated model that can accurately identify in-distribution (ID) and out-of-distribution (OOD) data for ensuring the training dataset quality. However, the core challenges for designing such as system are – (i) given the infinite variations of the anomaly, curation of training data is in-feasible; (ii) making assumptions about the types of anomalies are often hypothetical. The proposed work designed an unsupervised anomaly detection model using a cascade variational autoencoder coupled with a zero-shot learning network that maps the latent vectors to semantic attributes. The performance of the proposed model is shown on two different use cases – skin images and chest radiographs and also compare against the same class of state-of-the-art generative OOD detection models.
Details
Title
- Anomaly Detection using Cascade Variational Autoencoder Coupled with Zero Shot Learning – Medical Imaging Use Cases
Contributors
- Ramasamy, Gokul (Author)
- Banerjee, Imon (Thesis advisor)
- Sanyal, Arindam (Thesis advisor)
- Jayasuriya, Suren (Committee member)
- Arizona State University (Publisher)
Date Created
The date the item was original created (prior to any relationship with the ASU Digital Repositories.)
2023
Resource Type
Collections this item is in
Note
-
Partial requirement for: M.S., Arizona State University, 2023
-
Field of study: Electrical Engineering