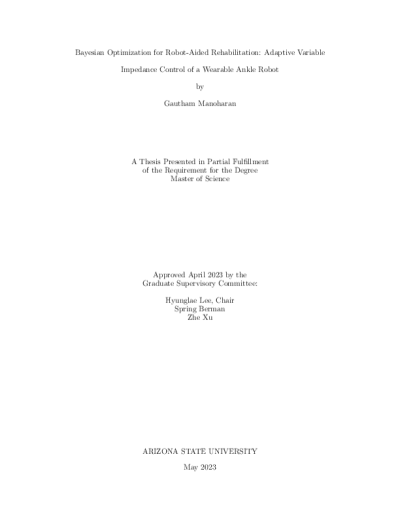
Description
This thesis presents a study on the user adaptive variable impedance control of a wearable ankle robot for robot-aided rehabilitation with a primary focus on enhancing accuracy and speed. The controller adjusts the impedance parameters based on the user's kinematic data to provide personalized assistance. Bayesian optimization is employed to minimize an objective function formulated from the user's kinematic data to adapt the impedance parameters per user, thereby enhancing speed and accuracy. Gaussian process is used as a surrogate model for optimization to account for uncertainties and outliers inherent to human experiments. Student-t process based outlier detection is utilized to enhance optimization robustness and accuracy. The efficacy of the optimization is evaluated based on measures of speed, accuracy, and effort, and compared with an untuned variable impedance controller during 2D curved trajectory following tasks. User effort was measured based on muscle activation data from the tibialis anterior, peroneus longus, soleus, and gastrocnemius muscles. The optimized controller was evaluated on 15 healthy subjects and demonstrated an average increase in speed of 9.85% and a decrease in deviation from the ideal trajectory of 7.57%, compared to an unoptimized variable impedance controller. The strategy also reduced the time to complete tasks by 6.57%, while maintaining a similar level of user effort.
Details
Title
- Bayesian Optimization for Robot-Aided Rehabilitation: Adaptive Variable Impedance Control of a Wearable Ankle Robot
Contributors
- Manoharan, Gautham (Author)
- Lee, Hyunglae (Thesis advisor)
- Berman, Spring (Committee member)
- Xu, Zhe (Committee member)
- Arizona State University (Publisher)
Date Created
The date the item was original created (prior to any relationship with the ASU Digital Repositories.)
2023
Subjects
Resource Type
Collections this item is in
Note
- Partial requirement for: M.S., Arizona State University, 2023
- Field of study: Electrical Engineering