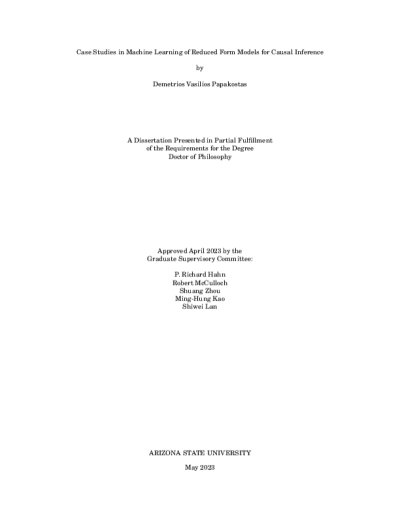
Description
This dissertation develops versatile modeling tools to estimate causal effects when conditional unconfoundedness is not immediately satisfied. Chapter 2 provides a brief overview ofcommon techniques in causal inference, with a focus on models relevant to the data explored
in later chapters. The rest of the dissertation focuses on the development of novel “reduced
form” models which are designed to assess the particular challenges of different datasets.
Chapter 3 explores the question of whether or not forecasts of bankruptcy cause bankruptcy.
The question arises from the observation that companies issued going concern opinions were
more likely to go bankrupt in the following year, leading people to speculate that the opinions themselves caused the bankruptcy via a “self-fulfilling prophecy”. A Bayesian machine
learning sensitivity analysis is developed to answer this question. In exchange for additional
flexibility and fewer assumptions, this approach loses point identification of causal effects
and thus a sensitivity analysis is developed to study a wide range of plausible scenarios of
the causal effect of going concern opinions on bankruptcy. Reported in the simulations are
different performance metrics of the model in comparison with other popular methods and a
robust analysis of the sensitivity of the model to mis-specification. Results on empirical data
indicate that forecasts of bankruptcies likely do have a small causal effect.
Chapter 4 studies the effects of vaccination on COVID-19 mortality at the state level in
the United States. The dynamic nature of the pandemic complicates more straightforward
regression adjustments and invalidates many alternative models. The chapter comments on
the limitations of mechanistic approaches as well as traditional statistical methods to epidemiological data. Instead, a state space model is developed that allows the study of the
ever-changing dynamics of the pandemic’s progression. In the first stage, the model decomposes the observed mortality data into component surges, and later uses this information in
a semi-parametric regression model for causal analysis. Results are investigated thoroughly
for empirical justification and stress-tested in simulated settings.
Details
Title
- Case Studies in Machine Learning of Reduced Form Models for Causal Inference
Contributors
- Papakostas, Demetrios (Author)
- Hahn, Paul (Thesis advisor)
- McCulloch, Robert (Committee member)
- Zhou, Shuang (Committee member)
- Kao, Ming-Hung (Committee member)
- Lan, Shiwei (Committee member)
- Arizona State University (Publisher)
Date Created
The date the item was original created (prior to any relationship with the ASU Digital Repositories.)
2023
Subjects
Resource Type
Collections this item is in
Note
- Partial requirement for: Ph.D., Arizona State University, 2023
- Field of study: Mathematics